|
ACCESS THE FULL ARTICLE
No SPIE Account? Create one
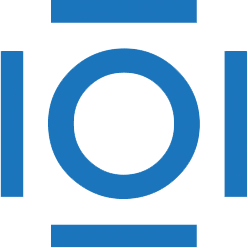
CITATIONS
Cited by 1 scholarly publication.
Long wavelength infrared
Mining
Statistical analysis
Numerical analysis
Databases
Signal to noise ratio
Calibration