|
ACCESS THE FULL ARTICLE
No SPIE Account? Create one
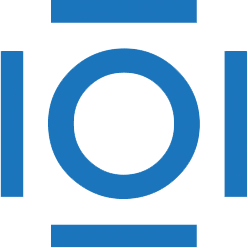
CITATIONS
Cited by 6 scholarly publications.
Solid state lighting
Image classification
Earth observing sensors
Remote sensing
Landsat
Statistical modeling
Binary data