|
ACCESS THE FULL ARTICLE
No SPIE Account? Create one
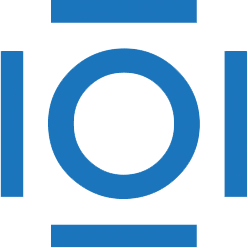
CITATIONS
Cited by 9 scholarly publications.
Reconstruction algorithms
Synthetic aperture radar
Scattering
Compressed sensing
Denoising
Radar imaging
Image denoising