|
ACCESS THE FULL ARTICLE
No SPIE Account? Create one
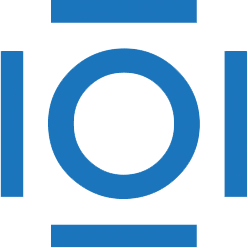
CITATIONS
Cited by 7 scholarly publications.
Earth observing sensors
Agriculture
Vegetation
Landsat
Reflectivity
Satellites
Satellite imaging