|
ACCESS THE FULL ARTICLE
No SPIE Account? Create one
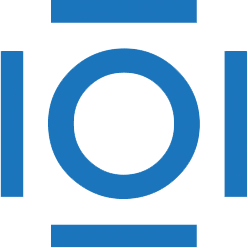
CITATIONS
Cited by 1 scholarly publication.
Data modeling
Data analysis
Algorithm development
Hyperspectral imaging
Neural networks
Remote sensing
Genetic algorithms