How to fully capture high-frequency information is an important issue in the remote sensing image super-resolution (SR) task. Most of the existing convolutional neural network based methods usually apply the attention mechanism to capture the high-frequency information. However, it is often insufficient since the remote sensing images usually contain more high-frequency information than natural images. Recently, some studies try to transform the original image into the wavelet domain to capture more high-frequency information. However, we observe that these methods usually apply similar network structures to learn different wavelet components, which will be difficult to fully capture the different features. To solve this issue, we propose a method named multi-frequency parallel wavelet transform network (MFPWTN) for remote sensing image SR. Specifically, we initially design two different network structures to reconstruct the high-frequency and low-frequency wavelet components, which can fully capture the characteristics of different frequencies. Subsequently, we introduce a high-frequency fusion module to enhance the information transmission among different high-frequency wavelet components. In addition, we employ the dilated convolution to establish the network structure for reconstructing the low-frequency wavelet component, which allows us to capture different receptive fields by using relatively few parameters. The experimental results on two public remote sensing datasets, UCMerced-LandUse and NWPU-RESISC45, show that the proposed MFPWTN can get superior performance over many existing state-of-the-art algorithms. |
ACCESS THE FULL ARTICLE
No SPIE Account? Create one
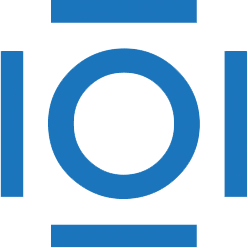
CITATIONS
Cited by 1 scholarly publication.
Wavelets
Image restoration
Remote sensing
Wavelet transforms
Convolution
Lawrencium
Feature fusion