|
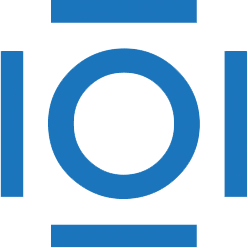
CITATIONS
Cited by 16 scholarly publications.
Hyperspectral imaging
Image classification
Prototyping
Fuzzy logic
Neurons
Data modeling
Fuzzy systems