|
ACCESS THE FULL ARTICLE
No SPIE Account? Create one
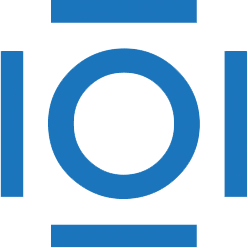
CITATIONS
Cited by 5 scholarly publications.
Hyperspectral imaging
Remote sensing
Image classification
Image fusion
Source mask optimization
Alternate lighting of surfaces
Detection and tracking algorithms