|
ACCESS THE FULL ARTICLE
No SPIE Account? Create one
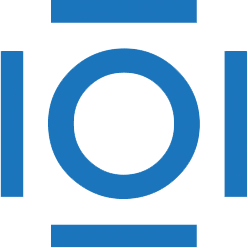
CITATIONS
Cited by 3 scholarly publications.
Convolutional neural networks
Network architectures
Target recognition
RGB color model
Video
Particle filters
Statistical analysis