Flame detection is critical to human society. Current flame detection methods have problems about huge model volume and large parameters. We proposed an improved YOLOv5-light that reduces the parameters while maintaining the same detection accuracy. First, we use the channel separation shuffling method to ensure the information exchange between channels and optimize the residual structure. Second, depthwise separable convolution is used as the downsampling module in the backbone network to reduce the model’s parameters further. Finally, the Ghost module is used to improve the feature extraction module in the head network to reduce computational cost caused by ordinary convolution. The final experimental results show that the proposed YOLOv5-light network achieves detection accuracy similar to that of YOLOv5, with a 65.6% decrease in parameters and a model volume of only 34.8% of the latter. |
ACCESS THE FULL ARTICLE
No SPIE Account? Create one
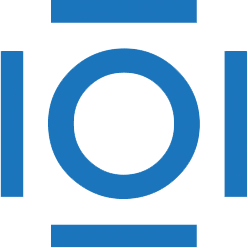
CITATIONS
Cited by 3 scholarly publications.
Flame detectors
Flame
Convolution
Feature extraction
Target detection
Convolutional neural networks
Object detection