|
ACCESS THE FULL ARTICLE
No SPIE Account? Create one
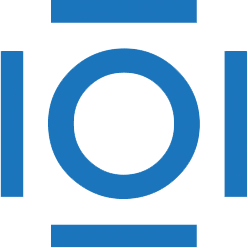
CITATIONS
Cited by 69 scholarly publications.
Image segmentation
Prostate
Magnetic resonance imaging
Diffusion weighted imaging
Convolution
Convolutional neural networks
Prostate cancer