|
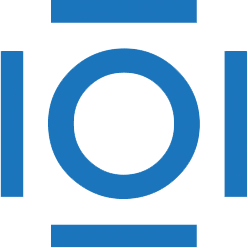
CITATIONS
Cited by 10 scholarly publications.
Brain
Simulation of CCA and DLA aggregates
Neuroimaging
Canonical correlation analysis
Genetics
Functional magnetic resonance imaging
Brain imaging