Lithography process hotspot is a traditional design and quality issue for the integrated circuit manufacturing due to the gap between exposure wavelength and critical feature size. To efficiently detect the hotspot regions and minimize the necessity of conducting expensive lithography simulation experiments, various pattern-based methods have been proposed in the past years. Recent solutions have been focused on implementing deep learning strategies because of the unique strength in imagery classification tasks by employing the artificial neural networks. However, solving the technical bottlenecks such as imbalanced learning, identifying rare hotspots and effective feature extraction remains challenging. For this research, we introduce a hotspot detection method based on a convolutional neural network classifier and enhanced it by the imagery feature extraction and a generative adversarial network data augmentation system. Experimental results show competitive performance compared with the existing works. |
ACCESS THE FULL ARTICLE
No SPIE Account? Create one
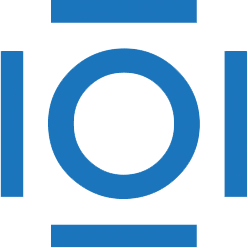
CITATIONS
Cited by 1 scholarly publication.
Lithography
Gallium nitride
Feature extraction
Manufacturing
Data modeling
Image processing
Photomasks