1.IntroductionIn the neurological intensive care unit (ICU), patients have pathophysiologic alterations in both systemic and intracranial physiology that require targeted interventions. Encased within the skull, diagnostic interrogations of brain physiology are more difficult compared to the rest of the body. The brain is particularly vulnerable to damage after ischemic and traumatic insults due to its high metabolic demands as well as the fact that it is enclosed within a rigid vault with little room to expand. Non-invasive, real-time monitors of brain function that can be deployed at the bedside are sorely needed. Near-infrared spectroscopy (NIRS) offers this possibility and has been studied in multiple forms of brain injury, including diffuse hypoxic-ischemic brain injury after cardiac arrest,1 subarachnoid hemorrhage (SAH),2,3 acute ischemic stroke,4,5 and traumatic brain injury (TBI).6,7 In this review, we will first discuss principles of cerebrovascular physiology and then discuss the features of and specific applications for NIRS in acute brain injuries. 2.Cerebral Blood Flow: Hemodynamic Autoregulation and Cerebrovascular ReactivityThe cerebrovascular network is structured to closely regulate cerebral blood flow (CBF) to ensure adequate perfusion. This is primarily controlled by vascular smooth muscle cells lining the pial and intraparenchymal arteries, which alter the vessel diameter and thus cerebrovascular resistance (CVR). Multiple mechanisms exist to modulate resistance and therefore CBF, including hemodynamic (myogenic) autoregulation and cerebrovascular carbon dioxide () reactivity. When these mechanisms are intact, the brain is adequately perfused and metabolic homeostasis is achieved. However, when neurovascular injury is sustained, these systems fail and subsequent vascular and metabolic derangements ensue. These derangements can lead to mismatches between cerebral metabolic demand and energy supply, ultimately causing further secondary brain injury. A major need in the ICU, therefore, is the ability to detect these vascular changes, and thereby make meaningful interventions to preserve and optimize cerebral hemodynamics. 2.1.Hemodynamic Autoregulation: Blood Pressure and Cerebral PerfusionThe cerebral vasculature reacts to changes in mean arterial pressure (MAP)—which ultimately affects vessel transmural pressure—by altering vessel diameter to regulate CBF.8,9 Changes in vessel diameter can alter intracranial pressure (ICP) through changes in cerebral blood volume, which ultimately can impact cerebral perfusion pressure (CPP). CPP is the major pressure gradient driving CBF (and therefore oxygen delivery) to cerebral tissue and can be crudely estimated by the equation CPP = MAP – ICP.10 [Fig. 1(a)]. It should be noted, however, that a more complete model requires consideration of the effects of vessel wall tension/tone11,12 in addition to ICP, on CPP. A more accurate equation for actual CPP (aCPP) is aCPP = MAP − CrCP, where CrCP is the “critical closing pressure.” CrCP is the arterial blood pressure at which CBF ceases due to its inability to overcome ICP and active wall tension in the small arterioles.13 CrCP can calculated by the linear slope of plotting MAP and CBF velocity and extrapolating the pressure at which velocity = 0.14 Fig. 1Major concepts in cerebral autoregulation and vascular reactivity. (a) Graph depicting relationship of CPP to CBF with intact cerebral autoregulation. Within the zone of intact autoregulation (dashed lines), changes in vessel diameter maintain consistent CBF. When CPP drops below the zone of autoregulation, ischemia ensues. Conversely, elevated pressures above the autoregulation zone lead to hyperemia and edema. (b) Optimal MAP () is calculated by comparing the cerebral TOI as measured by NIRS across the patient’s range of BP. The autoregulatory curve is superimposed in red, and the gray box denotes the patient’s MAP range that corresponds to those that fall within preserved autoregulation status (). corresponds to the nadir of the COx curve. (c) Cerebrovascular reactivity to is depicted with lower causing vasoconstriction. ![]() When autoregulatory mechanisms are intact, increases in CPP (caused either by increases in mean arterial blood pressure or decreases in ICP) are counteracted by vasoconstriction through the myogenic reflex, increasing CVR to maintain consistent CBF15 (Fig. 1). However, this commonly accepted heuristic may be an oversimplified view, given the wide individual differences in autoregulatory ranges as well as significant effects of sedative, anesthetic, and cardiovascular medications on autoregulation.16 In theory, autoregulation status can be measured by calculating the correlation between pressure (either CPP or MAP) and CBF over a range of spontaneously fluctuating pressures (positive correlation implies disrupted autoregulation, whereas absent correlation implies intact autoregulation). The correlation between MAP and ICP, a surrogate estimate of cerebral autoregulation, termed the cerebrovascular pressure reactivity index (PRx) has been validated as a marker of outcome in patients with multiple forms of brain injury.17 As a correlation coefficient, PRx values lie between and . Values closer to suggest worsening autoregulation status. PRx can be calculated over serial, overlapping time windows to provide a near real-time estimate of autoregulation status that is updated continuously. PRx values are generally interpreted to signify impaired autoregulation.18 Although PRx can provide valuable, clinically actionable data on autoregulation status, it is limited to patients receiving invasive ICP neuromonitoring. Transcranial Doppler (TCD) measures of CBF velocity can be recorded non-invasively; however, the use of TCD for continuous autoregulation measurement is practically hindered by the difficulty in performing continuous TCD recordings. Because of these issues, NIRS has emerged as an important tool for non-invasive bedside autoregulation assessment in the ICU. There are several NIRS-derived values that have been utilized to assess cerebral autoregulation. Early studies investigated the correlation between fluctuations in MAP and the difference between oxygenated and deoxygenated hemoglobin ([HbD] = [HbO] − [HbR]).19 This relative value is highly susceptible to artifacts.20 Alternative measures include (1) tissue oxygenation index (TOI), or the correlation between MAP and NIRS-derived measures of oxygenation calculated by the ratio of HbO/(HbO + HbR) multiplied by a scaling factor and expressed as a percentage and (2) cerebral oximetry index (COx), which is the correlation between MAP and NIRS-derived regional cerebral oxygen saturation ().20 These measures are less susceptible to movement artifacts. All these parameters function similarly to PRx in that the more negative the relationship between changes in MAP and measures of oxygenation are, the more intact the autoregulation is assumed to be.21 Alternatively, NIRS studies have also leveraged the presence of baseline low frequency oscillations in arterial blood pressure (ABP) that demonstrate NIRS correlates in the forms of oscillating changes to [HbO] and [HbR]; acute brain injuries (ABIs) have been shown to alter the oscillatory pattern and are thought to represent altered autoregulation.22 These approaches have been used to measure autoregulation status in patients with TBI,21 ischemic stroke,22–24 SAH,25 and diffuse hypoxic ischemic brain injury after cardiac arrest.26–28 Diffuse correlation spectroscopy (DCS) is a non-invasive diffuse optical technique that can provide a measure of relative CBF (rCBF) changes through detecting fluctuations in light intensity caused by red blood cells in the microvasculature (see Sec. 3.3), which allows for a direct comparison of ICP/CPP with CBF.29 In addition to measuring autoregulation status, NIRS can be utilized to identify an optimal MAP () or optimal CPP () where autoregulation status is ideal [Fig. 1(b)].18 Maintaining or could potentially limit secondary injury that occurs due to deviations in blood pressure (BP) that occur outside the range of optimal autoregulation. The general strategy is to serially measure an NIRS-based oxygenation index, such as COx or TOI, as well as a measure of perfusion, MAP or CPP. The blood/perfusion pressure where COx is at a minimum is taken to be (Fig. 2) or . A recent study investigated this strategy in patients with a variety of ABIs and found that greater absolute difference between clinically observed and optimal MAP was associated with increased risk of death.32 Fig. 2Examples of using NIRS derived autoregulation indices to identify . (a) Data from a pediatric cardiac arrest patient. Top graph shows the time course of fluctuations in MAP and over a 24-h period. Second from top, temporal fluctuations in COx are shown. Periods of time with poor autoregulation (i.e., ) are shaded gray. Second from bottom, method for deriving (MAP where COx is minimized) and both ULA and LLA (BP where COx > 0.3) is shown. Bottom graph shows temporal trends in superimposed on top of the patient’s actual BP. Areas shaded dark gray represent periods of time when actual MAP was significantly below (defined as periods where ). (b) Similar data from adult patients with non-traumatic subarachnoid hemorrhage. Black trace represents patient’s actual MAP, red trace represents trends in calculated from COx. Shaded red areas represent the range of intact autoregulation. The top curve shows a patient with BP trends where MAP largely stayed within ULA and LLA, whereas bottom curve shows a patient where BP often exceeded the ULA. [(a)- taken from Ref. 30 and (b)- taken from Ref. 31]. ![]() 2.2.Cerebrovascular Reactivity: -Regulated Vascular ChangesChemoreceptors in the cerebral vasculature induce vasodilation in response to increases in the partial pressure of ().33 This is thought to be related to -triggered release of vasoactive factors, such as nitric oxide, endothelin-1, and endothelial-derived hyperpolarizing factor from the vascular endothelium itself, and can be independent of changes in pH.34 Hyperventilation (which decreases ) constricts cerebral blood vessels and concomitantly can decrease ICP due to reductions in cerebral blood volume [Fig. 1(c)]. Cerebrovascular reactivity is compromised after brain injury, leading to patchy, asymmetric patterns of dilation in response to hypercapnic stimuli in which the healthy vessels dilate more readily, leading to preferentially greater regional CBF.35 Alterations in cerebrovascular reactivity are common in acute brain injury including TBI,35,36 SAH,37 and stroke,38,39 and have been shown to be poor prognostic indicators.37,40 Although blood oxygen level dependent functional magnetic resonance imaging (BOLD-fMRI) has been used previously to study cerebrovascular reactivity, magnetic resonance imaging (MRI) is a resource-intensive tool requiring significant time, support staff availability, and maintenance costs. Non-invasive assessment of cerebral autoregulation has been performed using TCDs in both healthy and critically ill patients by studying its relationship to systemic and respiratory CO2 levels.41,42 Specifically, different responses between end-tidal () levels to posterior cerebral artery and vertebral artery blood flow (BF) during exercise have been demonstrated,41 sugggesting that may have unique effects for different vessels throughout the brain. However, TCDs are limited by dependence on the technologist and difficulty with maintaining prolonged, continuous measurements. NIRS, in addition to its relatively low cost and portability, also demonstrates higher temporal resolution compared to functional MRI (fMRI) (albeit at the cost of spatial resolution) and can provide continuous assessments. Experimental animal studies using NIRS can directly show a significant correlation between the level of pCO2 changes and CBF.43 In addition, using NIRS in humans, a cerebrovascular reactivity index can be calculated by calculating the absolute change in HbO, HbR, and total hemoglobin (Hb)36,44,45 or alternatively 45,46 per 1 kPa increase in . reactivity measurements via fNIRS have been validated against both TCD44 and BOLD-fMRI,36,45 and demonstrate a significantly high correlation. Prior studies have utilized NIRS following various forms of both acute and chronic brain injury,47,48 as discussed further in each disease-specific application subsection below. levels can also influence autoregulation,49 and this interplay may be dysregulated in pathological states, such as sepsis.42 2.3.Neurovascular Coupling: Neural Activity-Induced Vascular ControlWith intact neurovascular coupling, cerebral blood vessels dilate in response to increased local energy or metabolic demands.50,51 Neurovascular coupling relies on neuronal activity-dependent release of chemical signals, such as prostaglandins, nitric oxide, and adenosine,51 to regulate and distribute CBF to the regions of highest neural activity. Because local BF responses during neurovascular coupling exceed metabolic demand, functional NIRS (fNIRS) can detect neurovascular coupling as a transient increase in both oxy- and total hemoglobin.52,53 ABIs can trigger inverse neurovascular coupling, where higher metabolic demand results in paradoxical vasoconstriction, depriving metabolically active brain tissue of oxygen and glucose delivery and potentiating neurotoxicity.54,55 This is most notably seen in association with cortical spreading depolarizations (CSDs), which are pathologic waves of extreme depolarization that emanate from areas of acute brain injury (see Sec. 5.3).55 3.Advantages and Disadvantages of NIRS and Related Technologies in the ICUThe limited absorption of near-infrared (NIR) range (650 to 950 nm) light through the skull provides the ability to non-invasively detect changes in hemodynamics and oxygenation in the brain tissue lying underneath. In addition, given its potential portability and high temporal resolution, diffuse optical imaging is potentially ideal for providing real-time measurements of cerebral physiology at the bedside. Multiple subcategories of non-invasive optical imaging provide distinct advantages and disadvantages, as discussed below (Table 1). Table 1Comparison of different NIRS methods and related technologies.
3.1.Continuous Wave-NIRSContinuous wave-NIRS (CW-NIRS) is the most used technology in commercial NIRS devices and in the ICU. It utilizes constant illumination of infrared light and measures changes in the absorption of the light that passes through the tissue to derive changes in HbO and HbR concentrations using the Modified Beer-Lambert Law (MBLL). CW-NIRS provides a measure of relative changes in hemodynamics and oxygenation and cannot quantify absolute HbO, HbR, and HbT concentrations.56,57 However, the portable nature and commercial availability of CW-NIRS systems makes them very attractive candidate devices for use at the bedside in the neonatal ICU (NICU). Commercial CW-NIRS devices generally utilize a multi-distance approach in which light is detected at different distances from the source to allow separation of superficial (e.g., scalp) signals from deeper signals in the brain.56 3.2.Frequency Domain-NIRS and Time Domain-NIRSFrequency domain (FD-)NIRS employs intensity-modulated illumination ( frequency) and phase-resolved detection, which measures not only the average intensity of the detected light but also its fluctuating amplitude and phase shift ().56,58 One important adantage of FD-NIRS is that it can provide quantitative measures of absolute HbO, HbR, and HbT as well as tissue oxygen status (, see Sec. 5.2 for clinical importance). Absolute measures allow better ability to compare data longitudinally within-subjects as well as between-subjects. In addition, at the modulation frequencies typically used in FD-NIRS (), recorded signals are less susceptible to ambient light, minimizing background artifacts.57,59,60 This may be particularly advantageous in the ICU where there are numerous potential artifacts from room lighting and stray light from other devices. In addition, the phase shift data derived from FD-NIRS also help to improve the signal-to-noise ratio as well as to improve depth sensitivity in cerebral tissue.61 The disadvantage to this technology is its large size, high cost, and paucity of commercially-available devices. Time domain (TD-)NIRS in contrast uses pulsed illumination and time-resolved detection. TD-NIRS devices emit an extremely short (), concentrated pulse of light into the tissue and measures the arrival times and paths of the photons that emerge from the tissue. The temporal distribution of the detected photons allows an assessment of the tissue’s optical properties (scattering and absorption); therefore, this technique also provides absolute hemodynamic/oxygenation data.56 This technology yields the highest amount of information, but it is also the most complex technology both for operation and analysis. As with FD-NIRS, there is a paucity of commercially available TD-NIRS devices approved for use in clinical settings, though at least one company has developed a portable bedside device that is similar in size to commercially available CW-NIRS.62 3.3.Diffuse Correlation SpectroscopyDCS is a related technology that uses coherent NIR-range light to measure hemodynamic changes in deep tissue microvasculature. It detects fluctuations in light intensity over time produced by mobile sources of scatter (primarily RBCs in the microvasculature) to produce a measure of rCBF known as a BF index (BFI),29 which is proportional to absolute CBF.63,64 It has excellent temporal resolution and penetrates through superficial tissue layers.65 DCS has been combined with NIRS in hybrid instruments to allow for absolute CBF measures, with NIRS used to calculate the DCS calibration coefficient , a constant that is dependent on tissue factors including the geometry and composition of regional microvasculature (e.g., arterial versus capillary versus venule) as well as tissue heterogeneity and optical properties, with tissue BFI.63,64 Improvement in determination of can be achieved by contrast-enhanced NIRS using injections of indocyanine green (ICG), a light-absorbing optical dye, and measuring its time-dependent absorption signal through tissue to provide absolute CBF at any given time point. The requirement of repeated ICG injections limits its use for continuous monitoring, and therefore other studies have created individualized patient calibration protocols to minimize this need.65 In addition, hybrid DCS/NIRS devices can simultaneously measure CBF and oxy-deoxy-hemoglobin concentrations, respectively,66–68 which allows for the derivation of metabolic rate of tissue oxygen consumption and evaluation of possible tissue ischemia (see Sec. 5.2). DCS has been validated against numerous gold-standard CBF techniques, including arterial spin labeling fMRI,69 TCD,70 and xenon-enhanced computed tomography.67 An advantage over these technologies in that DCS provides a continuous bedside assessment of absolute CBF. A disadvantage of DCS is the limited number of commercially available devices with no device currently cleared for clinical use. However, at least two bedside hybrid systems, one with DCS coupled with FD-NIRS71 and another with TD-NIRS,68 have become commercially available for research over the past few years. 3.3.1.Diffuse correlation tomographyWhile NIRS offers superior temporal resolution, a major limitation of NIRS is its poor spatial resolution. This presents a challenge for ABIs that classically involve more focal areas of injury. One advancement in DCS technology is diffuse correlation tomography (DCT), which can generate three-dimensional (3D) images of BF by analyzing CBF data from DCS using many source-detector pairs and a wide field of view (FOV) for image reconstruction. There are multiple approaches to DCT analysis (traditional versus speckle contrast), which are beyond the scope of this paper.72 Numerous animal studies have focused on the application of DCT to cerebral physiology, including in pharmacologically-induced CSDs,73 which are pathologic sequelae of many type of ABIs (see Sec. 5.3) and ischemia/stroke.74,75 This technology is limited by its susceptibility to noise and difficulty penetrating deeper tissues as would be required for CBF monitoring in humans.72 Moreover, speckle contrast DCT in particular requires a window (e.g., burr hole or craniotomy) for intracerebral monitoring.73 4.Applications of NIRS in Specific Brain InjuriesGiven the often profound impact of ABIs upon cerebral hemodynamics, NIRS and related technologies are powerful tools for assessment of patients’ cerebral autoregulation, vascular reactivity, and tissue oxygenation. To this end, many studies have utilized optical imaging to both diagnose focal/global injuries as well as to provide real-time data for optimizing clinical management (e.g., optimizing CPP). Below, we will discuss applications based upon particular forms of acute brain injury. 4.1.Sudden Cardiac ArrestOutcomes in survivors of sudden cardiac arrest are largely driven by the burden of hypoxic ischemic brain injury.76 Early studies using NIRS showed that a cerebral reduction of 20% or a level below 50% to 60% during induced ventricular fibrillation in humans is associated with ischemic brain injury.30 Cerebral oximetry has since been studied during both the initial resuscitation period as well as during subsequent post-arrest ICU care. In both adults and children, higher values measured during resuscitation are associated with higher rates of return of spontaneous circulation.77–79 One study in adults suggested that higher intra-arrest may also be associated with improved neurologic outcome.79 In the post-arrest period, NIRS has been utilized to measure the association between cerebral autoregulation state and neurologic outcome as well as to identify the . Studies in both adults and children have established the feasibility of using NIRS to identify individualized , which is generally higher than targets recommended in post-arrest care guidelines [Fig. 2(a)].26–28,80,81 In children, the overall burden of deviations in BP below is associated with increased risk of mortality.80 4.2.Extracorporeal Life SupportExtracorporeal life support is an important therapy used for high-risk cardiac surgeries [cardiopulmonary bypass (CPB)] as well as longer term support of patients with severe cardio-respiratory failure in the ICU [extracorporeal membrane oxygenation (ECMO)]. ABIs are common during CPB and ECMO due to both the high risk of thrombus formation within the extracorporeal circuit (which can lead to ischemic stroke) as well as the need for systemic anticoagulation (which can result in intracranial hemorrhage). NIRS-based cerebral oximetry has become a standard practice for monitoring brain function during open cardiac surgeries where CPB is used. Algorithms to optimize during surgery have been developed82 and shown to be feasible in reversing episodes of cerebral desaturation.1,83 In adults, a recent trial showed that using a strategy to optimize was associated with improved post-operative memory function; however, there was no difference in the burden of cerebral hypoxia between control and intervention groups.84 It remains unclear what aspects of the cerebral optimization strategy were responsible for the difference. In patients receiving ECMO, decreases in are associated with the development of ABIs (both acute ischemic strokes and intracranial hemorrhage)85,86 and worse outcomes.87 Further work is needed, however, to determine if cerebral oximetry monitoring is associated with improved outcomes. 4.3.Non-Traumatic Subarachnoid HemorrhageNon-traumatic SAH most often occurs as the result of the spontaneous rupture of a cerebral aneurysm.88 In addition to the primary brain injury caused by the initial bleeding event, SAH can trigger delayed cerebral ischemia (DCI) in the days that follow DCI. Multiple mechanisms—including cerebral vasospasm, microthrombosis, and CSD—mediate DCI, resulting in new ischemic infarcts that can occur anywhere between 3 and 4 days to more than 2 weeks after aneurysm rupture.89,90 TCD is routinely used clinically to screen for DCI but has only modest sensitivity and specificity.31,91 Studies have evaluated the ability of NIRS to identify both DCI (defined clinically and/or radiographically) as well as cerebral vasospasm (defined angiographically) after subarachnoid hemorrhage. Using TD-NIRS, one study showed acute drops in both and oxy-hemoglobin in eight patients, six of which had angiographically confirmed vasospasm.2 Another more recent study used CW-NIRS as a marker of DCI (defined clinically as a sustained decrease in Glasgow Coma Scale score of points or new focal neurologic deficit without other explainable cause) and showed that a reduction in of from baseline had 85.7% sensitivity and specificity for detecting DCI.92 In both studies, the accuracy of NIRS was superior to TCD. DCI is often preceded by impaired cerebral autoregulation that can be identified using NIRS or TCD days before onset.3,93 A recent study used both NIRS (COx) and ICP (PRx) based continuous autoregulation measurements to calculate optimal MAP and found that the burden of deviations away from individualized targets was associated with worse outcome [Fig. 2(b)].94 There was strong agreement between both COx and PRx based values. 4.4.Acute Ischemic StrokeIschemic stroke occurs after occlusion of a cerebral artery with resultant cessation of blood flow. Treatment revolves around re-opening the occlusion (either with pharmacologic thrombolysis, catheter directed endovascular thrombectomy, or a combination) in suitable candidates and optimizing cerebral perfusion to prevent infarct growth while limiting hemorrhagic complications in all patients. The traditional configuration of NIRS using forehead sensors monitors oxygenation in brain tissue supplied by the middle and anterior cerebral arteries. For this reason, NIRS has been studied during early revascularization therapies as a tool to monitor therapeutic success as well as during the subsequent stages of care. An observational study in 43 patients who received endovascular thrombectomy identified multiple types of desaturation events of potential clinical importance.4 Eleven patients showed distinct bilateral drops during endotracheal intubation. During the intervention, small peaks (more common) as well as sustained rises (less common) in were observed that correlated with recanalization. A greater interhemispheric difference at the end of the case was associated with mortality. A more recent study showed that successful recanalization was associated with a significant reduction in interhemispheric difference.5 NIRS has also been utilized to identify optimal MAP in the post-revascularization period using cerebral autoregulation measurements. During this time period, cerebral autoregulation is impaired, and vulnerable brain tissue surrounding the core infarct (termed the ischemic penumbra) is at risk for further ischemic damage (leading to expansion of total infarct volume) from hypoperfusion as well as hemorrhage from hyper/reperfusion injury. Petersen et al.95,96 calculated as well as upper and lower limits of autoregulation (ULA and LLA, respectively) and showed that that the burden of time spent above ULA had worse outcomes and higher risk of hemorrhagic transformation. Future trials are required to evaluate whether targeting and minimizing time spent above ULA lead to improved outcomes. 4.5.Traumatic Brain InjuryTBI is a leading cause of death and disability worldwide.97 Computed tomography (CT) imaging is the most frequently used diagnostic tool. Traumatic cerebrovascular injury, including SAH, subdural hematoma, epidural hematoma, and contusion/intraparenchymal hemorrhage, is often seen on CT, and the presence of different focal injuries can markedly affect management decisions. However, in low-resource, pre-hospital, and military/wartime settings, CT imaging is not readily available. Due to the resulting increased absorption of NIR light by dense areas of extravascular blood, NIRS can be potentially used for focal vascular injury detection. CW-NIRS allows relative Hb concentrations to be determined, with a comparison to the contralateral hemisphere or nearby “healthy” tissue.98 Lower scattering/high absorbance occurs in areas of hematoma. The commercially available Infrascanner™ is a portable, handheld device that uses this principle to detect hematomas cc in volume and from the pial surface. Studies demonstrate a sensitivity of hematoma detection between 78% and 93% and specificity 82.9% and 90% with positive predictive value (PPV) of 77% and negative predictive value (NPV) of 90%.6,7 An alternative device (CrainScan, BYTEC, Germany) that also uses CW-NIRS found similar efficacy in hematoma identification in a cohort of 148 patients. Out of the 54 CT-confirmed hematoma cases, NIRS detected 48 with a sensitivity of 88.9%, specificity 77.7%, PPV 69.6%, and NPV 62.8%.99 A theme throughout these studies is that due to the limitations of NIRS in penetrating far into the brain, more deeply seated lesions, such as those in the posterior fossa, remain occult. In addition, bilateral and small lesions also pose a challenge. FD-NIRS can be coupled with multiple optodes to create a 3D reconstruction of the target area, allowing localization of the lesion within a centimeter. This FD-diffuse optical tomography offers the potential for effective pre-hospitalization diagnosis and triage based on non-invasive imaging.100 However, they are larger in size, limiting their portability.98 In addition to focal lesions in TBI, more widespread microvascular injury is quite common and contributes to secondary injury. CPP optimization may limit the secondary damage that occurs from diffuse microvascular injury. Zwiefel and colleagues compared autoregulation indices measured by invasive ICP monitoring (PRx) and NIRS [total hemoglobin index (THx)] in 40 hospitalized subjects with TBI and found moderate overall correlation between the two indices (, ) that improved () after removing patients with frontal hematomas. Across all patients, and values were similar when calculated using PRx and THx.21 This paves the way for eventual non-invasive individualized optimization of cerebral hemodynamics, which may improve long-term patient outcomes. Finally, traumatic microvascular injury in TBI can lead to long term alterations in cerebrovascular reactivity to carbon dioxide. Amayot et al. explored the long-term effects of TBI on reactivity using a hypercapnia challenge using both BOLD-fMRI and NIRS. They found that in chronic TBI subjects, cerebrovascular reactivity was reduced both globally and to a greater extent focally (frontal regions) using both methods when compared to healthy controls.36 5.Challenges and Future Applications5.1.Non-Invasive Measurement of ICPGiven that the skull is a rigid container, the pressure within (ICP) is equal to the sum of the pressures exerted by its contents, which include the cerebral vasculature, brain parenchyma, and the cerebrospinal fluid (CSF).101 An increase in volume to any of these components will raise ICP, and if ICP surpasses critical values the risk of cerebral herniation markedly increases.101 In addition, since ICP is a key determinant of CPP, its continuous measurement is clinically valuable.15 ICP monitoring in general requires the placement of invasive probes directly into brain tissue, which requires neurosurgical expertise and carries the risk of associated infection and hemorrhage.102 Many patients cannot have invasive monitors placed due to higher risks of bleeding. Therfore, a non-invasive means of determining a patient’s ICP would have substantial clinical benefit. TCD103 and optic nerve sheath ultrasound104 have been explored as non-invasive methods to estimate ICP, but in both cases results can be highly variable and are examiner dependent.105 Likewise, imaging approaches with CT and MRI have not provided reliable estimates of ICP and are limited in feasbility due to radiation exposure and cost/time, respectively.105 Diffuse optics methods (including NIRS and DCS) may provide a viable non-invasive method for ICP measurement. Early studies have examined their use in non-human primate (NHP) models106,107 as well as in infants,108 by estimating ICP from the derived cardiac waveform106 and comparing this to the gold-standard invasive monitors. Importantly, alterations to relative [HbO] alone (which can be obtained with basic NIRS devices) appear to change with ICP and when used in combination with machine learning, can be used to derive ICP from waveform features in NHP with validation against invasive neuromonitors and CBF data from DCS.109 A different approach is to utilize DCS to measure CrCP, which is proportional to ICP but also factors in vasomotor tone. Although TCD has been shown to be capable of measuring CrCP non-invasively,110 it is limited by the ability to provide continuous measurements, technical anatomic challenges, and the risk of confounding hemodnamic facors, such as turbulent flow in large insonnated arteries.111 Baker et al.13 have demonstrated the ability to optically measure CrCP using DCS, thus bypassing these limitations. By assessing pulsatile CBF waveforms through the microvasculature, and comparing this to pulsatile peripheral arterial BP waveforms, DCS provided a highly reproducible, accurate method for measuring CrCP and thereby aCPP in healthy adults, which was validated against TCD.13 These initial results are promising and future studies in brain injured patient populations have the potential to lead to the development of powerful ICU tools. 5.2.Detection of Cerebral IschemiaTimely bedside identification of cerebral ischemia is a key principle in neurocritical care.112 This often requires placement of invasive, intraparenchymal monitors for measuring ICP, brain tissue oxygen tension (), CBF, and cerebral biochemistry. In general, invasive monitoring measures only surrogates for true ischemic injury and only samples small areas of injured tissue. In addition, using guideline endorsed physiological targets (e.g., , , ) does not guarantee that ischemic conditions are not occuring.112 Finally, many patients are not candidates for invasive monitor placement. Non-invasive global measures of physiology (e.g., MAP, ) cannot reliably reflect cerebral physiology. Given the high temporal resolution it offers, real-time non-invasive optical detection of critically-low tissue oxyenation levels via NIRS could facilitate meaningful clinical interventions and improve patient outcomes. In isolation, does not adequately measure tissue ischemia.113 However, in combination with cortical CBF indices (via optical technology, such as DCS)114–116 and arterial oxygen saturation, the oxygen extraction fraction and cerebral metabolic rate of oxygen can be determined and thereby can identify areas of perfusion-metabolic mismatch.115,117 This is a promising area for further studies in neurological disease states. 5.3.Non-Invasive Detection of CSDsPrior studies in pre-clinical models of TBI have demonstrated that not all neuronal damage occurs at the onset of trauma (the primary injury). Instead, subsequent, poorly-understood molecular and cellular mechanisms of secondary injury lead to ischemia and neurotoxicity, which are detrimental to functional outcomes following TBI.118 CSDs, first described by Leão119 as “cortical spreading depression”, represent one such potential mechanism. CSDs are slowly propagating (1 to ) waves of extreme depolarization followed by suppression of brain activity, which are common after acquired brain injury, including subarachnoid hemorrhage,120 ischemic stroke,121 and TBI.122 CSDs in TBI are notably associated with worse patient outcomes,123 and given their delayed nature, offer an appealing therapeutic target for prevention of secondary brain injury. However, real-time monitoring is needed to detect CSDs and titrate potential therapies; given that scalp electroencephalography (EEG) has not proven a reliable diagnostic tool for CSDs,124 their detection currently requires subdural electrocorticography. These challenges highlight the need for a non-invasive CSD detection method. Thought to result from mismatch between energy supply (e.g., CBF, metabolic substrates) and demand (metabolic rate),125 CSDs are associated with dramatic changes in neuronal and neurovascular function.55,126 In metabolically intact tissue, spreading depolarizations generally induce vasodilation. However, when the cerebral vasculature is compromised, CSDs commonly trigger a bimodal vascular response, consisting of initial vasoconstriction (i.e., inverse neurovascular coupling) resulting in reduced blood oxygenation, tissue hypoxia, and metabolic failure, followed by vasodilation. It is hypothesized that the deleterious effects of CSDs are in part due to these associated vascular manifestations.55 NIRS has been used to non-invasively detect neurovascular changes associated with CSD in migraines,127,128 ischemia in stroke,25 as well as cerebral dysregulation in TBI patients.21 CSDs produce dramatic changes in neurovascular dynamics, which have the potential to be detected via regional changes in CBF and metabolism as measured by NIRS. This is supported by studies using NIRS to detect spontaneous vascular changes associated with migrainous auras, which are widely thought to be secondary to CSDs.127 Because NIRS methods are non-invasive and compatible with continuous bedside monitoring in critically ill patients, this technology represents potential diagnostic and thereapeutic utility in the ICU. 5.4.Combined fNIRS and EEGCombining fNIRS with EEG, which evaluates the excitatory and inhibitory post-synaptic potentials generated from regional neural activity, offers the ability to evaluate neurovascular coupling. Although EEG and fNIRS have been used to study various neurological disorders, including seizures/epilepsy (e.g., delineating non-epileptic events from seizures129) and stroke (e.g., response to neurotherapeutic approaches in neurorehabilitation),54,130–132 there has been limited use in neurocritical care patients. However, given the important role of altered neurovascular coupling in acute forms of brain injury,54,55 the ability to detect and better understand this pathophysiology would be valuable in the ICU. Early studies in NHP models have demonstrated that there is a potential relationship between CPP/autoregulation and neurovascular coupling.133 In an NHP communicating hydrocephalus model, exogenous CSF was introducted into the animals ventricles, with CPP measured at each CSF volume. Concurrent EEG and fNIRS were applied to measure neural and vascular, respectively, evoked potentials during a visual stimulus exposure, and a resulting hemodynamic response function (HRF) was dervied as a measure of neurovascular coupling. As CPP became more deranged from physiologic values, the shape of the HRF, and therefore the status of neurovascular coupling, became more altered, independent from the subject’s .133 A better understanding of this mechanism and extension of these EEG-NIRS studies into ICU patients could allow for better CPP optimization post-brain injury. 5.5.Quantifying Patient/Caregiver Interactions and Identifying Covert ConsciousnessfNIRS has been applied to social interactions in what is termed “hyperscanning.” Classically, this involves a dyad (e.g., parent-child or two competitors in a game) undergoing simultaneous fNIRS recordings, with one instrument’s optodes divided between paricipants. This allows simultaneous interpersonal interactions and their resulting hemodynamic changes to be evaluated in real-time.134 Synchronized patterns provide information about various social neuroscience areas of interest, including competition and deception,135,136 cooperation,137,138 group communication,139 and childhood socialization/bonding.140 A potential future application of hyperscanning in the NICU lies in assessing patient-family/provider interactions in cases of post-injury coma. Given the “black box” that the comatose brain represents, there is much interest in identifying any covert consciousness that may be present. It is conceiveable that patients with better prognosis for functional recovery may have subtle synchronization descovered with concurrent patient-family or patient-provider fNIRS recordings. This would offer assistance with neuroprognostication and therefore guidance to family members. 6.ConclusionNIRS is emerging as a tool that can non-invasively monitor brain function and impact therapeutic decisions for patients admitted to the ICU with acute brain injuries. A growing body of literature suggests roles for identifying patients that develop or are at risk for developing secondary brain injury as well as optimizing hemodynamics based on an individual patient’s autoregulation status. Moreover, recent work in NIRS offers the possibility to non-invasively measure ICP and CrCP to provide important intervenable physiologic metrics.13,109 Advanced NIRS techniques, such as DCS, enable real-time measurements of absolute CBF141 and cerebral metabolism142 as well as improved ability to define areas of true cerebral ischemia.116 In addition, combining NIRS with other bedside tools, such as EEG, may improve its diagnostic accuracy.143 Future studies will be required to validate the impact of NIRS on patient outcomes. Finally, evaluating inter-brain synchronization using fNIRS hyperscanning may enable the study of otherwise unmeasurable cognitive interactions between unresponsive patients in the ICU and persons that interact with them (such as family members/caregivers and health care providers).144,145 Given its advantages of portability, non-invasiveness, and low-cost, NIRS will likely become more widely utilized by intensivists in the future. ReferencesA. Deschamps et al.,
“Cerebral oximetry monitoring to maintain normal cerebral oxygen saturation during high-risk cardiac surgery: a randomized controlled feasibility trial,”
Anesthesiology, 124 826
–836 https://doi.org/10.1097/ALN.0000000000001029 ANESAV 0003-3022
(2016).
Google Scholar
N. Yokose et al.,
“Bedside monitoring of cerebral blood oxygenation and hemodynamics after aneurysmal subarachnoid hemorrhage by quantitative time-resolved near-infrared spectroscopy,”
World Neurosurg, 73 508
–513 https://doi.org/10.1016/j.wneu.2010.02.061
(2010).
Google Scholar
K. P. Budohoski et al.,
“Cerebral autoregulation after subarachnoid hemorrhage: comparison of three methods,”
J. Cereb. Blood Flow Metab., 33 449
–456 https://doi.org/10.1038/jcbfm.2012.189
(2013).
Google Scholar
C. Hametner et al.,
“Noninvasive cerebral oximetry during endovascular therapy for acute ischemic stroke: an observational study,”
J. Cereb. Blood Flow Metab., 35 1722
–1728 https://doi.org/10.1038/jcbfm.2015.181
(2015).
Google Scholar
T. Ritzenthaler et al.,
“Cerebral near-infrared spectroscopy: a potential approach for thrombectomy monitoring,”
Stroke, 48 3390
–3392 https://doi.org/10.1161/STROKEAHA.117.019176 SJCCA7 0039-2499
(2017).
Google Scholar
H. Ayaz et al.,
“Early diagnosis of traumatic intracranial hematomas,”
J. Biomed. Opt., 24 051411 https://doi.org/10.1117/1.JBO.24.5.051411 JBOPFO 1083-3668
(2019).
Google Scholar
R. J. Brogan et al.,
“Near-infrared spectroscopy (NIRS) to detect traumatic intracranial haematoma: a systematic review and meta-analysis,”
Brain Inj., 31 581
–588 https://doi.org/10.1080/02699052.2017.1287956 BRAIEO 1362-301X
(2017).
Google Scholar
W. M. Bayliss,
“On the local reactions of the arterial wall to changes of internal pressure,”
J. Physiol., 28 220
–231 https://doi.org/10.1113/jphysiol.1902.sp000911 JPHYA7 0022-3751
(1902).
Google Scholar
D. W. Busija and D. D. Heistad,
“Factors involved in the physiological regulation of the cerebral circulation,”
Rev. Physiol. Biochem. Pharmacol., 101 161
–211 https://doi.org/10.1007/BFb0027696 RPBEA5 0303-4240
(1984).
Google Scholar
P. Le Roux,
“Physiological monitoring of the severe traumatic brain injury patient in the intensive care unit,”
Curr. Neurol Neurosci. Rep., 13 331 https://doi.org/10.1007/s11910-012-0331-2
(2013).
Google Scholar
S. Permutt and R. L. Riley,
“Hemodynamics of collapsible vessels with tone: the vascular waterfall,”
J. Appl. Physiol., 18 924
–932 https://doi.org/10.1152/jappl.1963.18.5.924
(1963).
Google Scholar
R. C. Dewey, H. P. Pieper and W. E. Hunt,
“Experimental cerebral hemodynamics. Vasomotor tone, critical closing pressure, and vascular bed resistance,”
J. Neurosurg., 41 597
–606 https://doi.org/10.3171/jns.1974.41.5.0597 JONSAC 0022-3085
(1974).
Google Scholar
W. B. Baker et al.,
“Noninvasive optical monitoring of critical closing pressure and arteriole compliance in human subjects,”
J. Cereb. Blood Flow Metab., 37 2691
–2705 https://doi.org/10.1177/0271678X17709166
(2017).
Google Scholar
R. Aaslid et al.,
“Dynamic pressure—flow velocity relationships in the human cerebral circulation,”
Stroke, 34 1645
–1649 https://doi.org/10.1161/01.STR.0000077927.63758.B6 SJCCA7 0039-2499
(2003).
Google Scholar
N. A. Lassen,
“Cerebral blood flow and oxygen consumption in man,”
Physiol. Rev., 39 183
–238 https://doi.org/10.1152/physrev.1959.39.2.183 PHREA7 0031-9333
(1959).
Google Scholar
P. Brassard et al.,
“Losing the dogmatic view of cerebral autoregulation,”
Physiol. Rep., 9 e14982 https://doi.org/10.14814/phy2.14982
(2021).
Google Scholar
M. Czosnyka et al.,
“Continuous assessment of the cerebral vasomotor reactivity in head injury,”
Neurosurgery, 41 11
–17 https://doi.org/10.1097/00006123-199707000-00005 NEQUEB
(1997).
Google Scholar
L. Rivera-Lara et al.,
“Cerebral autoregulation-oriented therapy at the bedside: a comprehensive review,”
Anesthesiology, 126 1187
–1199 https://doi.org/10.1097/ALN.0000000000001625 ANESAV 0003-3022
(2017).
Google Scholar
M. Tsuji et al.,
“Cerebral intravascular oxygenation correlates with mean arterial pressure in critically ill premature infants,”
Pediatrics, 106 625
–632 https://doi.org/10.1542/peds.106.4.625 PEDIAU 0031-4005
(2000).
Google Scholar
A. Caicedo et al.,
“Cerebral tissue oxygenation and regional oxygen saturation can be used to study cerebral autoregulation in prematurely born infants,”
Pediatr. Res., 69 548
–553 https://doi.org/10.1203/PDR.0b013e3182176d85 PEREBL 0031-3998
(2011).
Google Scholar
C. Zweifel et al.,
“Noninvasive monitoring of cerebrovascular reactivity with near infrared spectroscopy in head-injured patients,”
J. Neurotrauma, 27 1951
–1958 https://doi.org/10.1089/neu.2010.1388 JNEUE4 0897-7151
(2010).
Google Scholar
A. V. Andersen et al.,
“Assessing low-frequency oscillations in cerebrovascular diseases and related conditions with near-infrared spectroscopy: a plausible method for evaluating cerebral autoregulation?,”
Neurophotonics, 5 030901 https://doi.org/10.1117/1.NPh.5.3.030901
(2018).
Google Scholar
Z. Li et al.,
“Wavelet analysis of cerebral oxygenation signal measured by near infrared spectroscopy in subjects with cerebral infarction,”
Microvasc. Res., 80 142
–147 https://doi.org/10.1016/j.mvr.2010.02.004 MIVRA6 0026-2862
(2010).
Google Scholar
D. Phillip et al.,
“Altered low frequency oscillations of cortical vessels in patients with cerebrovascular occlusive disease - a NIRS study,”
Front. Neurol., 4 204 https://doi.org/10.3389/fneur.2013.00204
(2013).
Google Scholar
C. Zweifel et al.,
“Continuous assessment of cerebral autoregulation with near-infrared spectroscopy in adults after subarachnoid hemorrhage,”
Stroke, 41 1963
–1968 https://doi.org/10.1161/STROKEAHA.109.577320 SJCCA7 0039-2499
(2010).
Google Scholar
K. Ameloot et al.,
“An observational near-infrared spectroscopy study on cerebral autoregulation in post-cardiac arrest patients: time to drop ‘one-size-fits-all’ hemodynamic targets?,”
Resuscitation, 90 121
–126 https://doi.org/10.1016/j.resuscitation.2015.03.001 RSUSBS 0300-9572
(2015).
Google Scholar
D. E. G. Griesdale et al.,
“Near-infrared spectroscopy to assess cerebral autoregulation and optimal mean arterial pressure in patients with hypoxic-ischemic brain injury: a prospective multicenter feasibility study,”
Crit. Care Explor., 2 e0217 https://doi.org/10.1097/CCE.0000000000000217
(2020).
Google Scholar
P. Pham et al.,
“Are changes in cerebrovascular autoregulation following cardiac arrest associated with neurological outcome? Results of a pilot study,”
Resuscitation, 96 192
–198 https://doi.org/10.1016/j.resuscitation.2015.08.007 RSUSBS 0300-9572
(2015).
Google Scholar
C. Cheung et al.,
“In vivo cerebrovascular measurement combining diffuse near-infrared absorption and correlation spectroscopies,”
Phys. Med. Biol., 46 2053
–2065 https://doi.org/10.1088/0031-9155/46/8/302 PHMBA7 0031-9155
(2001).
Google Scholar
W. J. Levy, S. Levin and B. Chance,
“Near-infrared measurement of cerebral oxygenation. Correlation with electroencephalographic ischemia during ventricular fibrillation,”
Anesthesiology, 83 738
–746 https://doi.org/10.1097/00000542-199510000-00013 ANESAV 0003-3022
(1995).
Google Scholar
E. Carrera et al.,
“Transcranial Doppler for predicting delayed cerebral ischemia after subarachnoid hemorrhage,”
Neurosurgery, 65 316
–323 https://doi.org/10.1227/01.NEU.0000349209.69973.88 NEQUEB
(2009).
Google Scholar
L. Rivera-Lara et al.,
“Optimizing mean arterial pressure in acutely comatose patients using cerebral autoregulation multimodal monitoring with near-infrared spectroscopy,”
Crit. Care Med., 47 1409
–1415 https://doi.org/10.1097/CCM.0000000000003908 CCMDC7 0090-3493
(2019).
Google Scholar
A. Battisti-Charbonney, J. Fisher and J. Duffin,
“The cerebrovascular response to carbon dioxide in humans,”
J. Physiol., 589 3039
–3048 https://doi.org/10.1113/jphysiol.2011.206052 JPHYA7 0022-3751
(2011).
Google Scholar
S. Yoon, M. Zuccarello and R. M. Rapoport,
“pCO(2) and pH regulation of cerebral blood flow,”
Front. Physiol., 3 365 https://doi.org/10.3389/fphys.2012.00365 FROPBK 0301-536X
(2012).
Google Scholar
F. Amyot et al.,
“Imaging of cerebrovascular function in chronic traumatic brain injury,”
J. Neurotrauma, 35 1116
–1123 https://doi.org/10.1089/neu.2017.5114 JNEUE4 0897-7151
(2018).
Google Scholar
F. Amyot et al.,
“Assessment of cerebrovascular dysfunction after traumatic brain injury with fMRI and fNIRS,”
Neuroimage Clin., 25 102086 https://doi.org/10.1016/j.nicl.2019.102086
(2020).
Google Scholar
J. Klingelhofer and D. Sander,
“Doppler test as an indicator of cerebral vasoreactivity and prognosis in severe intracranial hemorrhages,”
Stroke, 23 962
–966 https://doi.org/10.1161/01.str.23.7.962 SJCCA7 0039-2499
(1992).
Google Scholar
P. Hedera, P. Traubner and J. Bujdakova,
“Short-term prognosis of stroke due to occlusion of internal carotid artery based on transcranial Doppler ultrasonography,”
Stroke, 23 1069
–1072 https://doi.org/10.1161/01.STR.23.8.1069 SJCCA7 0039-2499
(1992).
Google Scholar
F. Vernieri et al.,
“Near infrared spectroscopy and transcranial Doppler in monohemispheric stroke,”
Eur. Neurol., 41 159
–162 https://doi.org/10.1159/000008041
(1999).
Google Scholar
W. Schalen, K. Messeter and C. H. Nordstrom,
“Cerebral vasoreactivity and the prediction of outcome in severe traumatic brain lesions,”
Acta Anaesthesiol. Scand., 35 113
–122 https://doi.org/10.1111/j.1399-6576.1991.tb03258.x
(1991).
Google Scholar
T. Washio et al.,
“Site-specific different dynamic cerebral autoregulation and cerebrovascular response to carbon dioxide in posterior cerebral circulation during isometric exercise in healthy young men,”
Auton. Neurosci., 238 102943 https://doi.org/10.1016/j.autneu.2022.102943
(2022).
Google Scholar
I. A. Crippa, A. Alvaro Quispe Cornejo and F. S. Taccone,
“Changes in arterial carbon dioxide partial pressure do not affect cerebral autoregulation in septic patients,”
Neurocrit. Care, 37 572
–574 https://doi.org/10.1007/s12028-022-01502-6
(2022).
Google Scholar
F. Silvera et al.,
“Study of the relationship between regional cerebral saturation and changes during mechanical ventilation to evaluate modifications in cerebral perfusion in a newborn piglet model,”
Braz. J. Med. Biol. Res., 55 e11543 https://doi.org/10.1590/1414-431x2022e11543 BJMRDK 0100-879X
(2022).
Google Scholar
P. Smielewski et al.,
“Can cerebrovascular reactivity be measured with near-infrared spectroscopy?,”
Stroke, 26 2285
–2292 https://doi.org/10.1161/01.STR.26.12.2285 SJCCA7 0039-2499
(1995).
Google Scholar
T. Alderliesten et al.,
“Simultaneous quantitative assessment of cerebral physiology using respiratory-calibrated MRI and near-infrared spectroscopy in healthy adults,”
Neuroimage, 85
(Pt 1), 255
–263 https://doi.org/10.1016/j.neuroimage.2013.07.015 NEIMEF 1053-8119
(2014).
Google Scholar
S. Miller and K. Mitra,
“NIRS-based cerebrovascular regulation assessment: exercise and cerebrovascular reactivity,”
Neurophotonics, 4 041503 https://doi.org/10.1117/1.NPh.4.4.041503
(2017).
Google Scholar
K. Kenney et al.,
“Phosphodiesterase-5 inhibition potentiates cerebrovascular reactivity in chronic traumatic brain injury,”
Ann. Clin. Transl. Neurol., 5 418
–428 https://doi.org/10.1002/acn3.541
(2018).
Google Scholar
A. Oldag et al.,
“Near-infrared spectroscopy and transcranial sonography to evaluate cerebral autoregulation in middle cerebral artery steno-occlusive disease,”
J. Neurol., 263 2296
–2301 https://doi.org/10.1007/s00415-016-8262-5
(2016).
Google Scholar
L. Meng and A. W. Gelb,
“Regulation of cerebral autoregulation by carbon dioxide,”
Anesthesiology, 122 196
–205 https://doi.org/10.1097/ALN.0000000000000506 ANESAV 0003-3022
(2015).
Google Scholar
C. Huneau, H. Benali and H. Chabriat,
“Investigating human neurovascular coupling using functional neuroimaging: a critical review of dynamic models,”
Front. Neurosci., 9 467 https://doi.org/10.3389/fnins.2015.00467 1662-453X
(2015).
Google Scholar
A. A. Phillips et al.,
“Neurovascular coupling in humans: physiology, methodological advances and clinical implications,”
J. Cereb. Blood Flow Metab., 36 647
–664 https://doi.org/10.1177/0271678X15617954
(2016).
Google Scholar
H. Obrig,
“NIRS in clinical neurology - a ‘promising’ tool?,”
Neuroimage, 85
(Pt 1), 535
–546 https://doi.org/10.1016/j.neuroimage.2013.03.045 NEIMEF 1053-8119
(2014).
Google Scholar
M. A. Yucel et al.,
“Functional near infrared spectroscopy: enabling routine functional brain imaging,”
Curr. Opin. Biomed. Eng., 4 78
–86 https://doi.org/10.1016/j.cobme.2017.09.011
(2017).
Google Scholar
J. Zhang et al.,
“Altered coupling between cerebral blood flow and voxel-mirrored homotopic connectivity affects stroke-induced speech comprehension deficits,”
Front. Aging Neurosci., 14 922154 https://doi.org/10.3389/fnagi.2022.922154
(2022).
Google Scholar
J. M. Hinzman et al.,
“Inverse neurovascular coupling to cortical spreading depolarizations in severe brain trauma,”
Brain, 137 2960
–2972 https://doi.org/10.1093/brain/awu241 BRAIAK 0006-8950
(2014).
Google Scholar
F. Scholkmann et al.,
“A review on continuous wave functional near-infrared spectroscopy and imaging instrumentation and methodology,”
Neuroimage, 85
(Pt 1), 6
–27 https://doi.org/10.1016/j.neuroimage.2013.05.004 NEIMEF 1053-8119
(2014).
Google Scholar
S. Fantini et al.,
“Non-invasive optical monitoring of the newborn piglet brain using continuous-wave and frequency-domain spectroscopy,”
Phys. Med. Biol., 44 1543
–1563 https://doi.org/10.1088/0031-9155/44/6/308 PHMBA7 0031-9155
(1999).
Google Scholar
W. L. Chen et al.,
“Functional near-infrared spectroscopy and its clinical application in the field of neuroscience: advances and future directions,”
Front. Neurosci., 14 724 https://doi.org/10.3389/fnins.2020.00724 1662-453X
(2020).
Google Scholar
M. Wolf et al.,
“Absolute frequency-domain pulse oximetry of the brain: methodology and measurements,”
Adv. Exp. Med. Biol., 530 61
–73 https://doi.org/10.1007/978-1-4615-0075-9_7 AEMBAP 0065-2598
(2003).
Google Scholar
S. Fantini and A. Sassaroli,
“Frequency-domain techniques for cerebral and functional near-infrared spectroscopy,”
Front. Neurosci., 14 300 https://doi.org/10.3389/fnins.2020.00300 1662-453X
(2020).
Google Scholar
M. Doulgerakis, A. T. Eggebrecht and H. Dehghani,
“High-density functional diffuse optical tomography based on frequency-domain measurements improves image quality and spatial resolution,”
Neurophotonics, 6 035007 https://doi.org/10.1117/1.NPh.6.3.035007
(2019).
Google Scholar
M. Lacerenza et al.,
“Wearable and wireless time-domain near-infrared spectroscopy system for brain and muscle hemodynamic monitoring,”
Biomed. Opt. Express, 11 5934
–5949 https://doi.org/10.1364/BOE.403327 BOEICL 2156-7085
(2020).
Google Scholar
R. C. Mesquita et al.,
“Direct measurement of tissue blood flow and metabolism with diffuse optics,”
Philos. Trans. A Math. Phys. Eng. Sci., 369 4390
–4406 https://doi.org/10.1098/rsta.2011.0232
(2011).
Google Scholar
D. A. Boas et al.,
“Establishing the diffuse correlation spectroscopy signal relationship with blood flow,”
Neurophotonics, 3 031412 https://doi.org/10.1117/1.NPh.3.3.031412
(2016).
Google Scholar
L. He et al.,
“Noninvasive continuous optical monitoring of absolute cerebral blood flow in critically ill adults,”
Neurophotonics, 5 045006 https://doi.org/10.1117/1.NPh.5.4.045006
(2018).
Google Scholar
T. Durduran et al.,
“Transcranial optical monitoring of cerebrovascular hemodynamics in acute stroke patients,”
Opt. Express, 17 3884
–3902 https://doi.org/10.1364/OE.17.003884 OPEXFF 1094-4087
(2009).
Google Scholar
M. N. Kim et al.,
“Noninvasive measurement of cerebral blood flow and blood oxygenation using near-infrared and diffuse correlation spectroscopies in critically brain-injured adults,”
Neurocrit. Care, 12 173
–180 https://doi.org/10.1007/s12028-009-9305-x
(2010).
Google Scholar
M. Giovannella et al.,
“BabyLux device: a diffuse optical system integrating diffuse correlation spectroscopy and time-resolved near-infrared spectroscopy for the neuromonitoring of the premature newborn brain,”
Neurophotonics, 6 025007 https://doi.org/10.1117/1.NPh.6.2.025007
(2019).
Google Scholar
G. Yu et al.,
“Validation of diffuse correlation spectroscopy for muscle blood flow with concurrent arterial spin labeled perfusion MRI,”
Opt. Express, 15 1064
–1075 https://doi.org/10.1364/OE.15.001064 OPEXFF 1094-4087
(2007).
Google Scholar
E. M. Buckley et al.,
“Cerebral hemodynamics in preterm infants during positional intervention measured with diffuse correlation spectroscopy and transcranial Doppler ultrasound,”
Opt. Express, 17 12571
–12581 https://doi.org/10.1364/OE.17.012571 OPEXFF 1094-4087
(2009).
Google Scholar
S. A. Carp et al.,
“Combined multi-distance frequency domain and diffuse correlation spectroscopy system with simultaneous data acquisition and real-time analysis,”
Biomed. Opt. Express, 8 3993
–4006 https://doi.org/10.1364/BOE.8.003993 BOEICL 2156-7085
(2017).
Google Scholar
M. X. Mireles et al., Biomedical Optical Imaging: From Nanoscopy to Tomography, AIP Publishing, Melville, New York, United States
(2021). Google Scholar
C. Zhou et al.,
“Diffuse optical correlation tomography of cerebral blood flow during cortical spreading depression in rat brain,”
Opt. Express, 14 1125
–1144 https://doi.org/10.1364/OE.14.001125 OPEXFF 1094-4087
(2006).
Google Scholar
C. Huang et al.,
“Noninvasive noncontact speckle contrast diffuse correlation tomography of cerebral blood flow in rats,”
Neuroimage, 198 160
–169 https://doi.org/10.1016/j.neuroimage.2019.05.047 NEIMEF 1053-8119
(2019).
Google Scholar
C. Huang et al.,
“Speckle contrast diffuse correlation tomography of cerebral blood flow in perinatal disease model of neonatal piglets,”
J Biophotonics, 14 e202000366 https://doi.org/10.1002/jbio.202000366
(2021).
Google Scholar
R. W. Neumar et al.,
“Post-cardiac arrest syndrome: epidemiology, pathophysiology, treatment, and prognostication. A consensus statement from the International Liaison Committee on Resuscitation (American Heart Association, Australian and New Zealand Council on Resuscitation, European Resuscitation Council, Heart and Stroke Foundation of Canada, InterAmerican Heart Foundation, Resuscitation Council of Asia, and the Resuscitation Council of Southern Africa); the American Heart Association Emergency Cardiovascular Care Committee; the Council on Cardiovascular Surgery and Anesthesia; the Council on Cardiopulmonary, Perioperative, and Critical Care; the Council on Clinical Cardiology; and the Stroke Council,”
Circulation, 118 2452
–2483 https://doi.org/10.1161/CIRCULATIONAHA.108.190652 CIRCAZ 0009-7322
(2008).
Google Scholar
C. Francoeur et al.,
“Near-infrared spectroscopy during cardiopulmonary resuscitation for pediatric cardiac arrest: a prospective, observational study,”
Resuscitation, 174 35
–41 https://doi.org/10.1016/j.resuscitation.2022.03.014 RSUSBS 0300-9572
(2022).
Google Scholar
A. W. Ibrahim et al.,
“Cerebral oximetry as a real-time monitoring tool to assess quality of in-hospital cardiopulmonary resuscitation and post cardiac arrest care,”
J. Am. Heart Assoc., 4 e001859 https://doi.org/10.1161/JAHA.115.001859
(2015).
Google Scholar
S. Parnia et al.,
“Cerebral oximetry during cardiac arrest: a multicenter study of neurologic outcomes and survival,”
Crit. Care Med., 44 1663
–1674 https://doi.org/10.1097/CCM.0000000000001723 CCMDC7 0090-3493
(2016).
Google Scholar
M. P. Kirschen et al.,
“Deviations from NIRS-derived optimal blood pressure are associated with worse outcomes after pediatric cardiac arrest,”
Resuscitation, 168 110
–118 https://doi.org/10.1016/j.resuscitation.2021.09.023 RSUSBS 0300-9572
(2021).
Google Scholar
J. Laurikkala et al.,
“Association of deranged cerebrovascular reactivity with brain injury following cardiac arrest: a post-hoc analysis of the COMACARE trial,”
Crit. Care, 25 350 https://doi.org/10.1186/s13054-021-03764-6
(2021).
Google Scholar
A. Denault, A. Deschamps and J. M. Murkin,
“A proposed algorithm for the intraoperative use of cerebral near-infrared spectroscopy,”
Semin. Cardiothorac. Vasc. Anesth., 11 274
–281 https://doi.org/10.1177/1089253207311685
(2007).
Google Scholar
A. Deschamps et al.,
“Reversal of decreases in cerebral saturation in high-risk cardiac surgery,”
J. Cardiothorac. Vasc. Anesth., 27 1260
–1266 https://doi.org/10.1053/j.jvca.2013.01.019 JCVAEK 1053-0770
(2013).
Google Scholar
S. Uysal et al.,
“Optimizing cerebral oxygenation in cardiac surgery: a randomized controlled trial examining neurocognitive and perioperative outcomes,”
J. Thorac. Cardiovasc. Surg., 159 943
–953.e3 https://doi.org/10.1016/j.jtcvs.2019.03.036 JTCSAQ 0022-5223
(2020).
Google Scholar
M. F. Hunt et al.,
“The use of cerebral NIRS monitoring to identify acute brain injury in patients with VA-ECMO,”
J. Intensive Care Med., 36 1403
–1409 https://doi.org/10.1177/0885066620966962
(2021).
Google Scholar
S. Pozzebon et al.,
“Cerebral near-infrared spectroscopy in adult patients undergoing veno-arterial extracorporeal membrane oxygenation,”
Neurocrit. Care, 29 94
–104 https://doi.org/10.1007/s12028-018-0512-1
(2018).
Google Scholar
M. Vedrenne-Cloquet et al.,
“Association of cerebral oxymetry with short-term outcome in critically ill children undergoing extracorporeal membrane oxygenation,”
Neurocrit. Care, 35 409
–417 https://doi.org/10.1007/s12028-020-01179-9
(2021).
Google Scholar
R. L. Macdonald and T. A. Schweizer,
“Spontaneous subarachnoid haemorrhage,”
Lancet, 389 655
–666 https://doi.org/10.1016/S0140-6736(16)30668-7 LANCAO 0140-6736
(2017).
Google Scholar
W. S. Dodd et al.,
“Pathophysiology of delayed cerebral ischemia after subarachnoid hemorrhage: a review,”
J. Am. Heart Assoc., 10 e021845 https://doi.org/10.1161/JAHA.121.021845
(2021).
Google Scholar
R. L. Macdonald,
“Delayed neurological deterioration after subarachnoid haemorrhage,”
Nat. Rev. Neurol., 10 44
–58 https://doi.org/10.1038/nrneurol.2013.246
(2014).
Google Scholar
C. Lysakowski et al.,
“Transcranial Doppler versus angiography in patients with vasospasm due to a ruptured cerebral aneurysm: a systematic review,”
Stroke, 32 2292
–2298 https://doi.org/10.1161/hs1001.097108 SJCCA7 0039-2499
(2001).
Google Scholar
J. J. Park et al.,
“Application of near-infrared spectroscopy for the detection of delayed cerebral ischemia in poor-grade subarachnoid hemorrhage,”
Neurocrit. Care, 35 767
–774 https://doi.org/10.1007/s12028-021-01223-2
(2021).
Google Scholar
K. P. Budohoski et al.,
“Impairment of cerebral autoregulation predicts delayed cerebral ischemia after subarachnoid hemorrhage: a prospective observational study,”
Stroke, 43 3230
–3237 https://doi.org/10.1161/STROKEAHA.112.669788 SJCCA7 0039-2499
(2012).
Google Scholar
A. Silverman et al.,
“Deviation from personalized blood pressure targets is associated with worse outcome after subarachnoid hemorrhage,”
Stroke, 50 2729
–2737 https://doi.org/10.1161/STROKEAHA.119.026282 SJCCA7 0039-2499
(2019).
Google Scholar
N. H. Petersen et al.,
“Fixed compared with autoregulation-oriented blood pressure thresholds after mechanical thrombectomy for ischemic stroke,”
Stroke, 51 914
–921 https://doi.org/10.1161/STROKEAHA.119.026596 SJCCA7 0039-2499
(2020).
Google Scholar
N. H. Petersen et al.,
“Association of personalized blood pressure targets with hemorrhagic transformation and functional outcome after endovascular stroke therapy,”
JAMA Neurol., 76 1256
–1258 https://doi.org/10.1001/jamaneurol.2019.2120
(2019).
Google Scholar
A. I. R. Maas et al.,
“Traumatic brain injury: integrated approaches to improve prevention, clinical care, and research,”
Lancet Neurol., 16 987
–1048 https://doi.org/10.1016/S1474-4422(17)30371-X
(2017).
Google Scholar
M. D. Whiting et al.,
““Prehospital detection of life-threatening intracranial pathology: an unmet need for severe TBI in Austere, rural, and remote areas,”
Front. Neurol., 11 599268 https://doi.org/10.3389/fneur.2020.599268
(2020).
Google Scholar
H. Ghalenoui et al.,
“Near-infrared laser spectroscopy as a screening tool for detecting hematoma in patients with head trauma,”
Prehosp. Disaster Med., 23 558
–561 https://doi.org/10.1017/S1049023X00006415
(2008).
Google Scholar
M. Ferrari and V. Quaresima,
“A brief review on the history of human functional near-infrared spectroscopy (fNIRS) development and fields of application,”
Neuroimage, 63 921
–935 https://doi.org/10.1016/j.neuroimage.2012.03.049 NEIMEF 1053-8119
(2012).
Google Scholar
M. H. Wilson,
“Monro-Kellie 2.0: the dynamic vascular and venous pathophysiological components of intracranial pressure,”
J. Cereb. Blood Flow Metab., 36 1338
–1350 https://doi.org/10.1177/0271678X16648711
(2016).
Google Scholar
P. H. Raboel et al.,
“Intracranial pressure monitoring: invasive versus non-invasive methods-a review,”
Crit. Care Res. Pract., 2012 950393 https://doi.org/10.1155/2012/950393
(2012).
Google Scholar
D. Cardim et al.,
“Non-invasive monitoring of intracranial pressure using transcranial doppler ultrasonography: is it possible?,”
Neurocrit. Care, 25 473
–491 https://doi.org/10.1007/s12028-016-0258-6
(2016).
Google Scholar
C. Robba et al.,
“Optic nerve sheath diameter measured sonographically as non-invasive estimator of intracranial pressure: a systematic review and meta-analysis,”
Intensive Care Med., 44 1284
–1294 https://doi.org/10.1007/s00134-018-5305-7 ICMED9 0342-4642
(2018).
Google Scholar
J. B. Rosenberg et al.,
“Non-invasive methods of estimating intracranial pressure,”
Neurocrit. Care, 15 599
–608 https://doi.org/10.1007/s12028-011-9545-4
(2011).
Google Scholar
A. Ruesch et al.,
“Erratum: Estimating intracranial pressure using pulsatile cerebral blood flow measured with diffuse correlation spectroscopy: erratum,”
Biomed. Opt. Express, 13 710
–712 https://doi.org/10.1364/BOE.452731 BOEICL 2156-7085
(2022).
Google Scholar
A. Ruesch et al.,
“Fluctuations in intracranial pressure can be estimated non-invasively using near-infrared spectroscopy in non-human primates,”
J. Cereb. Blood Flow Metab., 40 2304
–2314 https://doi.org/10.1177/0271678X19891359
(2020).
Google Scholar
J. B. Fischer et al.,
“Non-invasive estimation of intracranial pressure by diffuse optics: a proof-of-concept study,”
J. Neurotrauma, 37 2569
–2579 https://doi.org/10.1089/neu.2019.6965 JNEUE4 0897-7151
(2020).
Google Scholar
F. A. J. Relander et al.,
“Using near-infrared spectroscopy and a random forest regressor to estimate intracranial pressure,”
Neurophotonics, 9 045001 https://doi.org/10.1117/1.NPh.9.4.045001
(2022).
Google Scholar
R. Aaslid et al.,
“Dynamic pressure-flow velocity relationships in the human cerebral circulation,”
Stroke, 34
(7), 1645
–1649 https://doi.org/10.1161/01.STR.0000077927.63758.B6
(2003).
Google Scholar
M. Soehle et al.,
“Critical closing pressure in subarachnoid hemorrhage: effect of cerebral vasospasm and limitations of a transcranial Doppler-derived estimation,”
Stroke, 35 1393
–1398 https://doi.org/10.1161/01.STR.0000128411.07036.a9 SJCCA7 0039-2499
(2004).
Google Scholar
D. O. Okonkwo et al.,
“Brain oxygen optimization in severe traumatic brain injury phase-II: a phase II randomized trial,”
Crit. Care Med., 45 1907
–1914 https://doi.org/10.1097/CCM.0000000000002619 CCMDC7 0090-3493
(2017).
Google Scholar
D. J. Davies et al.,
“Cerebral oxygenation in traumatic brain injury: can a non-invasive frequency domain near-infrared spectroscopy device detect changes in brain tissue oxygen tension as well as the established invasive monitor?,”
J. Neurotrauma, 36 1175
–1183 https://doi.org/10.1089/neu.2018.5667 JNEUE4 0897-7151
(2019).
Google Scholar
E. M. Buckley et al.,
“Diffuse correlation spectroscopy for measurement of cerebral blood flow: future prospects,”
Neurophotonics, 1 011009 https://doi.org/10.1117/1.NPh.1.1.011009
(2014).
Google Scholar
K. Verdecchia et al.,
“Quantifying the cerebral metabolic rate of oxygen by combining diffuse correlation spectroscopy and time-resolved near-infrared spectroscopy,”
J. Biomed. Opt., 18 027007 https://doi.org/10.1117/1.JBO.18.2.027007 JBOPFO 1083-3668
(2013).
Google Scholar
D. R. Busch et al.,
“Detection of brain hypoxia based on noninvasive optical monitoring of cerebral blood flow with diffuse correlation spectroscopy,”
Neurocrit. Care, 30 72
–80 https://doi.org/10.1007/s12028-018-0573-1
(2019).
Google Scholar
J. Selb et al.,
“Erratum: Prolonged monitoring of cerebral blood flow and autoregulation with diffuse correlation spectroscopy in neurocritical care patients,”
Neurophotonics, 7 019801 https://doi.org/10.1117/1.NPh.7.1.019801
(2020).
Google Scholar
K. Kenney et al.,
“Cerebral vascular injury in traumatic brain injury,”
Exp. Neurol., 275
(Pt 3), 353
–366 https://doi.org/10.1016/j.expneurol.2015.05.019 EXNEAC 0014-4886
(2016).
Google Scholar
A. A. Leao,
“Further observations on the spreading depression of activity in the cerebral cortex,”
J. Neurophysiol., 10 409
–414 https://doi.org/10.1152/jn.1947.10.6.409 JONEA4 0022-3077
(1947).
Google Scholar
M. Lauritzen et al.,
“Clinical relevance of cortical spreading depression in neurological disorders: migraine, malignant stroke, subarachnoid and intracranial hemorrhage, and traumatic brain injury,”
J. Cereb. Blood Flow Metab., 31 17
–35 https://doi.org/10.1038/jcbfm.2010.191
(2011).
Google Scholar
A. J. Strong et al.,
“Peri-infarct depolarizations lead to loss of perfusion in ischaemic gyrencephalic cerebral cortex,”
Brain, 130 995
–1008 https://doi.org/10.1093/brain/awl392 BRAIAK 0006-8950
(2007).
Google Scholar
J. A. Hartings et al.,
“Spreading depolarizations and late secondary insults after traumatic brain injury,”
J. Neurotrauma, 26 1857
–1866 https://doi.org/10.1089/neu.2009.0961 JNEUE4 0897-7151
(2009).
Google Scholar
J. A. Hartings et al.,
“Spreading depolarisations and outcome after traumatic brain injury: a prospective observational study,”
Lancet Neurol., 10 1058
–1064 https://doi.org/10.1016/S1474-4422(11)70243-5
(2011).
Google Scholar
J. Hofmeijer et al.,
“Detecting cortical spreading depolarization with full band scalp electroencephalography: an illusion?,”
Front. Neurol., 9 17 https://doi.org/10.3389/fneur.2018.00017
(2018).
Google Scholar
D. von Bornstadt et al.,
“Supply-demand mismatch transients in susceptible peri-infarct hot zones explain the origins of spreading injury depolarizations,”
Neuron, 85 1117
–1131 https://doi.org/10.1016/j.neuron.2015.02.007 NERNET 0896-6273
(2015).
Google Scholar
J. M. Hinzman et al.,
“Excitotoxicity and metabolic crisis are associated with spreading depolarizations in severe traumatic brain injury patients,”
J. Neurotrauma, 33 1775
–1783 https://doi.org/10.1089/neu.2015.4226 JNEUE4 0897-7151
(2016).
Google Scholar
S. Viola et al.,
“Pathophysiology of migraine attack with prolonged aura revealed by transcranial Doppler and near infrared spectroscopy,”
Neurol. Sci., 31
(Suppl. 1), 165
–166 https://doi.org/10.1007/s10072-010-0318-1
(2010).
Google Scholar
A. Pourshoghi et al.,
“Cerebral reactivity in migraine patients measured with functional near-infrared spectroscopy,”
Eur. J. Med. Res., 20 96 https://doi.org/10.1186/s40001-015-0190-9 EJMRDJ 0379-8399
(2015).
Google Scholar
A. Kassab et al.,
“Multichannel wearable fNIRS-EEG system for long-term clinical monitoring,”
Hum. Brain Mapp., 39 7
–23 https://doi.org/10.1002/hbm.23849 HBRME7 1065-9471
(2018).
Google Scholar
U. Jindal et al.,
“Corticospinal excitability changes to anodal tDCS elucidated with NIRS-EEG joint-imaging: an ischemic stroke study,”
in Annu. Int. Conf. IEEE Eng. Med. Biol. Soc.,
3399
–3402
(2015). https://doi.org/10.1109/EMBC.2015.7319122 Google Scholar
A. Dutta et al.,
“EEG-NIRS based assessment of neurovascular coupling during anodal transcranial direct current stimulation—a stroke case series,”
J. Med. Syst., 39 205 https://doi.org/10.1007/s10916-015-0205-7 JMSYDA 0148-5598
(2015).
Google Scholar
D. Yang, Y. I. Shin and K. S. Hong,
“Systemic review on transcranial electrical stimulation parameters and EEG/fNIRS features for brain diseases,”
Front. Neurosci., 15 629323 https://doi.org/10.3389/fnins.2021.629323 1662-453X
(2021).
Google Scholar
D. Acharya et al.,
“Changes in neurovascular coupling with cerebral perfusion pressure indicate a link to cerebral autoregulation,”
J. Cereb. Blood Flow Metab., 42 1247
–1258 https://doi.org/10.1177/0271678X221076566
(2022).
Google Scholar
F. Babiloni and L. Astolfi,
“Social neuroscience and hyperscanning techniques: past, present and future,”
Neurosci. Biobehav. Rev., 44 76
–93 https://doi.org/10.1016/j.neubiorev.2012.07.006 NBREDE 0149-7634
(2014).
Google Scholar
M. Piva et al.,
“Distributed neural activity patterns during human-to-human competition,”
Front. Hum. Neurosci., 11 571 https://doi.org/10.3389/fnhum.2017.00571
(2017).
Google Scholar
M. Zhang et al.,
“Gender difference in spontaneous deception: a hyperscanning study using functional near-infrared spectroscopy,”
Sci. Rep., 7 7508 https://doi.org/10.1038/s41598-017-06764-1 SRCEC3 2045-2322
(2017).
Google Scholar
Y. Pan et al.,
“Cooperation in lovers: an fNIRS-based hyperscanning study,”
Hum. Brain Mapp., 38 831
–841 https://doi.org/10.1002/hbm.23421 HBRME7 1065-9471
(2017).
Google Scholar
N. Osaka et al.,
“How two brains make one synchronized mind in the inferior frontal cortex: fNIRS-based hyperscanning during cooperative singing,”
Front. Psychol., 6 1811 https://doi.org/10.3389/fpsyg.2015.01811 1664-1078
(2015).
Google Scholar
T. Nozawa et al.,
“Interpersonal frontopolar neural synchronization in group communication: an exploration toward fNIRS hyperscanning of natural interactions,”
Neuroimage, 133 484
–497 https://doi.org/10.1016/j.neuroimage.2016.03.059 NEIMEF 1053-8119
(2016).
Google Scholar
V. Reindl et al.,
“Brain-to-brain synchrony in parent-child dyads and the relationship with emotion regulation revealed by fNIRS-based hyperscanning,”
Neuroimage, 178 493
–502 https://doi.org/10.1016/j.neuroimage.2018.05.060 NEIMEF 1053-8119
(2018).
Google Scholar
D. Milej et al.,
“Quantification of cerebral blood flow in adults by contrast-enhanced near-infrared spectroscopy: validation against MRI,”
J. Cereb. Blood Flow Metab., 40 1672
–1684 https://doi.org/10.1177/0271678X19872564
(2020).
Google Scholar
W. B. Baker et al.,
“Continuous non-invasive optical monitoring of cerebral blood flow and oxidative metabolism after acute brain injury,”
J. Cereb. Blood Flow Metab., 39 1469
–1485 https://doi.org/10.1177/0271678X19846657
(2019).
Google Scholar
R. Li et al.,
“Concurrent fNIRS and EEG for brain function investigation: a systematic, methodology-focused review,”
Sensors (Basel), 22 5865 https://doi.org/10.3390/s22155865
(2022).
Google Scholar
A. Czeszumski et al.,
“Hyperscanning: a valid method to study neural inter-brain underpinnings of social interaction,”
Front. Hum. Neurosci., 14 39 https://doi.org/10.3389/fnhum.2020.00039
(2020).
Google Scholar
F. Scholkmann et al.,
“A new methodical approach in neuroscience: assessing inter-personal brain coupling using functional near-infrared imaging (fNIRI) hyperscanning,”
Front. Hum. Neurosci., 7 813 https://doi.org/10.3389/fnhum.2013.00813
(2013).
Google Scholar
BiographyRachel Thomas received her MD and PhD degrees at the University of Texas Southwestern Medical Center and is currently a neurology resident at the University of Pennsylvania. She is currently investigating the utility of NIRS and DCS to non-invasively measure cerebral autoregulation and spreading depolarizations after traumatic brain injury. Samuel S. Shin is an assistant professor of neurology at the University of Pennsylvania. He received his MD and PhD degrees from the University of Pittsburgh. He completed his Neurology residency at Johns Hopkins University followed by neurocritical care fellowship at the University of Pennsylvania. His research focuses on both large animal model and clinical studies of traumatic brain injury. Ramani Balu is an attending physician in neurocritical care at Inova Fairfax Hospital and adjunct assistant professor of neurology at the University of Pennsylvania. He received his MD and PhD degrees from Case Western Reserve University, followed by a neurology residency and neurocritical care fellowship at the University of Pennsylvania. He has investigated the use of NIRS and DCS to measure cerebral metabolism and blood flow in patients with acute brain injury. |
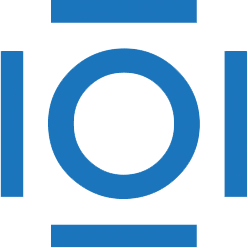
CITATIONS
Cited by 3 scholarly publications.
Near infrared spectroscopy
Traumatic brain injury
Injuries
Tissues
Brain
Hemodynamics
Oxygenation