1.IntroductionFunctional near-infrared spectroscopy (fNIRS) is a neuroimaging technique that uses the principle of neuro-vascular coupling to estimate the blood oxygen-level dependent (BOLD) response as a surrogate for neural activation and deactivation.1 Brain oxygenation is measured through light-emitting and receiving optodes using two different wavelengths within the “optical window” of 700 to 900 nm differentiating between oxygenated () and deoxygenated hemoglobin (HHb) absorption.2,3 fNIRS is a non-invasive and user-friendly technique, which has been validated against functional magnetic resonance imaging.4 Among the drawbacks of fNIRS are its limited spatial resolution and cortical penetration.5 However, fNIRS systems are mobile and less sensitive to motion artifacts than other mobile neuroimaging systems, such as electroencephalography (EEG),6 allowing for measurements during actual whole-body movements in natural environments.7 For these reasons, fNIRS is becoming increasingly popular for investigating the cortical activation patterns during postural and gait-related tasks.5,8 In addition, more and more intervention studies are implementing fNIRS as a primary outcome, comparing cortical activation before and after training in repeated measures designs.9–13 For example, studies have used fNIRS to investigate learning-induced neural changes in the prefrontal cortex (PFC) as well as in other motor regions during balance9,12,13 and manual tasks10,11 in young adults. These studies, however, did not take test-retest reliability into account, crucial for interpreting the changes in the BOLD signal as being meaningful rather than signifying measurement error. There are several potential sources of error, including the limited spatial specificity of fNIRS,14 as well as the systemic changes in physiological measures that may arise during movement due to heart rate and blood flow alterations.15 Other possible inaccuracies may arise from day-to-day variability in hemodynamic oscillations and whether the fNIRS-cap was repositioned precisely by the operator.16 So far, fNIRS test-retest reliability has shown to be fair to excellent during resting-state.17–19 Seven studies investigated fNIRS test-retest reliability of the PFC or the contralateral motor cortex during motor tasks, based on the intraclass correlation coefficient (ICC).20–24 The ICC provides an overall estimate of the correlation and agreement between measurements. It is calculated as the proportion of between-subject variance over the total variance.25 Four studies reported good to excellent reliability during manual tasks (), of which two studied task-specific motor channels ().20,22 One study reported very poor test-retest reliability () during passive hand movements imposed by a robot.21 Only two studies investigated test-retest reliability during gait-related tasks,26,27 showing fair to good reliability for straight walking (;26 27) and turning (27) in the PFC in young and middle-aged adults. None of these studies were conducted in older adults, who may present lower signal to noise ratios.28 Two studies pertained to patients with multiple sclerosis26 and traumatic brain injury,23 resulting in poor () and good () reliability in the PFC. Given the above-described gaps in the literature, we set out to investigate the test-retest reliability of fNIRS in healthy older adults during two different motor tasks, namely a postural weight-shifting and a finger tapping task. First, test-retest reliability of five regions of interest (ROIs) was determined during a weight-shifting task comparing two tests, which were repeated twice on the same day and on two consecutive days following cap removal and repositioning. Next, the task-specificity of test-retest reliability was investigated during a control finger tapping task, focusing on the contralateral finger region of the motor cortex.29 Based on earlier work during both gross and fine motor tasks in young adults26,27 and the anticipated lower signal to noise ratios in older people,28 we hypothesized that test–retest reliability would be more compromised than that obtained in young adults. Furthermore, we expected lower reliability after cap removal compared to when the cap remained stationary. 2.Materials and Methods2.1.ParticipantsHealthy older adults were recruited via an existing local database, compliant with the general data protection regulation (GDPR), as part of a larger randomized controlled trial (RCT; clinicaltrial.gov ID: NCT04594148). Participants had to be at least 65 years old, right handed (self-reported) and be able to independently stand upright for at least 5 min. Participants were excluded if they had a self-reported history of neurological disorders, balance impairments (i.e., vestibular disorders), uncorrected visual impairment, chronic musculoskeletal problems (e.g., osteoarthritis, osteoporosis), cardiovascular (e.g., uncontrolled hypertension, peripheral vascular disease) or respiratory (e.g., chronic obstructive pulmonary disease) disease, or diabetes related polyneuropathy. Additionally, participants with a cognitive impairment (Montreal cognitive assessment ) were excluded. Written informed consent was obtained from all participants prior to enrolment. The study was approved by the Ethics Committee Research UZ/KU Leuven (study ID: S62917). 2.2.Experimental Procedure and Tasks2.2.1.ProcedureOnly participants who were randomized to the passive control group of the RCT, who received no intervention, were included. Participants were assessed on 2 consecutive days. Motor tests were assessed twice on day 1, before and after a resting period of 30 min while keeping the fNIRS cap and optodes in place. After a complete removal of the fNIRS system, the cap was re-attached the next day and the third test was conducted around the same time of day as the first measurement the day before. Cap position across days was standardized (see Sec. 2.2.4). Motor and fNIRS assessments were conducted using a block design with each block consisting of seven trials of 20 sec of rest followed by 20 sec of movement. Prior pilot testing showed an 80% true positive rate when using this design. The start of each trial was marked in the fNIRS signal with a task-synchronized trigger. The 20-sec resting period, conducted in stance for the postural task and in sitting for the tapping task, served as a baseline for the following movement trial. The postural task blocks were conducted before the tapping task in a fixed order. 2.2.2.Postural taskThe postural task consisted of a non-immersive virtual reality weight-shifting task,30 as described in detail elsewhere (see Figure S1 in the Supplementary Material).31 In short, participants were standing in front of a screen at approximately three meters distance. They were instructed to shift their weight mediolaterally of their a-priori determined individual limits of stability, which activated a virtual water jet. With the water jet, they attempted to hit as many virtual wasps as possible, which appeared on the left and right side of the screen alternately. Participants’ center of mass (CoM) was captured within Nexus software (Vicon, Oxford Metrics, United Kingdom) by recording reflective markers placed bilaterally on the acromia, posterior superior iliac spines, lateral epicondyles, and lateral malleoli. Calculation of the CoM was done online within the D-Flow software (Motek Medical BV, Amsterdam, The Netherlands; version 3.28), based on the formulation by Winter (2009).32 During rest, subjects were asked to stand and watch the screen, displaying a video recording of the wasp game. 2.2.3.Finger tapping taskThe finger-tapping task, as part of the cloud-UPDRS application (version 1.3.0),33 was performed with the right dominant hand on a smartphone. It consisted of two visual targets with a diameter of 0.6 inch, positioned at a 2-inch distance from each other (see Figure S2 in the Supplementary Material). Participants were seated on a chair in front of the smartphone placed on a table. They were instructed to alternately tap the left and right targets with their right index finger while holding the smartphone still with their left hand. To prevent any learning effect, tapping frequency was set at 180 beats per minute imposed by a metronome beat. The researcher verbally indicated the start and termination of the task. Participants were instructed to sit as still as possible and place their right hand flat on the table during the 20-sec resting periods in between tapping trials. 2.2.4.fNIRS assessmentBrain hemodynamics were recorded following recent consensus guidelines.5 A continuous wave, single-phase fNIRS system (NIRSport2, NIRx, Berlin, Germany), using light emitting diodes (LEDs) with wavelengths of 760 and 850 nm at a sampling frequency of 7.81 Hz, recorded brain hemodynamics within the Aurora software (version 2020.7). First, participants’ head circumference was measured and matched to the closest corresponding cap size, varying from 54 to 60 cm. The Cz anatomical landmark was then determined using the inion, nasion, and pre-auricular points after which the lightweight cap was carefully placed on the head. To ensure similar cap placement on day two, the Cz, CP2, and FC1 anatomical landmarks were marked. Participants were asked not to wash their hair in between measurement days. A total of 32 optodes (16 sources, 16 detectors) were used to cover predefined ROIs, including the prefrontal cortex (PFC; Brodmann areas 9, 10, and 46), frontal eye fields (FEF; Brodmann area 8), supplementary motor area (SMA; Brodmann area 6, medial), premotor cortex (PMC; Brodmann area 6, lateral), and somatosensory cortex (SSC; Brodmann areas 1, 3, 5 and 7). The 10-10 layout in the fNIRS Optodes’ Location Decider (fOLD) toolbox34 was used to specify the optode locations (see Table S1 in the Supplementary Material) with a source-detector separation of . Sixteen short-separation channels with a source-detector separation of 8 mm were included, one over each source, to correct for physiological noise in the fNIRS signal.35 Furthermore, two accelerometers were placed at the back of the cap to correct for movement artefacts related to head movements. At the onset of testing, signal quality was visually checked and improved by moving the hair aside from underneath the optodes, if needed. An additional opaque cap was then placed over the fNIRS set-up to protect external light from interfering with the hemodynamic measurements. For the tapping task, the motor channel in between optode C3-C1 of the contralateral (left) hemisphere was chosen as the ROI. As the fOLD toolbox does not provide specific information on anatomical brain representations, the C3-C1 hand motor channel was based on the EEG topography36 in accordance with previous data on the homunculus hand position.37 2.3.Data Processing2.3.1.Behavioral dataWeight-shifting data were exported by D-Flow and analyzed within MATLAB 2018b (MathWorks, Natick, Massachusetts, United States). Similar to our previous analysis,31 CoM data were first low-pass filtered with a fourth-order Butterworth filter (cut-off = 6 Hz). Weight-shifting was then determined as the movement from the 80% stability limits threshold on the right to the 80% stability limits threshold on the left and vice versa. Outcome measures included weight-shifting speed and accuracy (CoM error) averaged over the seven trials. The cloud-UPDRS smartphone data were analyzed with Microsoft Excel (version 2016). Outcome measures included the average number of taps per trial and the accuracy of target taps in pixels (target error) and calculated as: where Xposition and Yposition refer to the coordinates of the finger on the screen, and Xtarget and Ytarget refer to the coordinates of the targets on the screen.2.3.2.fNIRS dataBrain hemodynamic data were analyzed with the open access NIRS toolbox ( https://github.com/huppertt/nirs-toolbox)38 implemented in MATLAB 2018b (MathWorks, Natick, Massachusetts, United States). Raw intensity signals were checked visually, resampled to 5 Hz, and converted into optical density using the Beer-Lambert law and a partial path length correction factor of 0.1, thereby correcting for light scatter caused by the brain tissue that the near-infrared light was travelling though, as stated in previous research.39,40 A general linear model, including short separation channels and accelerometers, was used to estimate the task hemodynamic response, thereby correcting for signal variations due to physiological noise and movement artefacts.20,35 The autoregressive iteratively reweighted least squares method was implemented to correct for motion and auto correlated noise.41 This method, including short separation channel regression, outperformed other filtering methods35 and was shown to improve data reproducibility.35 Accelerometers identified and corrected for changes in the fNIRS signal that corresponded with the accelerometer signal over a time-window of 15 s.42 Channels were averaged within the predefined ROIs. A channel was included if the spatial specificity, as determined with the fOLD toolbox,34 was at least 50% within a ROI43 (see Fig. 1). As the SMA and PMC ROIs were grouped within the fOLD output, the medial channels were defined as SMA and the lateral channels as PMC.44 The primary motor cortex (M1) was not included as ROI in this analysis, because spatial specificity did not exceed 50%. Midline-traversing channels were excluded, as cerebrospinal fluid running through the superior sagittal sinus could have interfered with the measurement of the underlying brain hemodynamics.45 In addition, the tapping task-specific hand motor channel C3-C1 was excluded from the ROIs specified for the postural task, as spatial specificity was lower than 50% (35% M1 and 35% PMC). Trial-averaged relative oxygenated hemoglobin ( active trial - rest trial ()) was used as the primary outcome for each ROI. As secondary outcomes, HHb () and () values for the left and right ROIs were investigated separately. Fig. 1fNIRS lay-out with ROIs according to the fOLD toolbox for the postural task with a specificity level . The PFC is represented by the blue colored channels, the FEF by the green channels, the SMA by the orange channels, the PMC by the purple channels, and the SSC by the yellow channels. The channels situated at the midline were excluded (indicated by black crosses). The C3-C1 channel (black circle; brown channel) was included as the task-specific hand motor channel for the finger tapping task, consistent with the EEG topography. Nz, naison; Iz, inion; LPA, left point auricular; and RPA, right point auricular. ![]() To a-posteriori check which channels were active during the postural or the finger-tapping task, signal changes in individual channels were assessed in a complementary analysis. A channel was classified as active when there was a significant average change during the task compared to rest in both (positive change) and HHb levels (negative change) over the three time points, which was FDR-corrected for the number of channels. 2.4.Statistical AnalysisStatistical analyses were performed with SPSS (IBM SPSS Statistics, version 26). After checking the data distribution, test-retest differences in behavioral performance on both the postural and tapping task were investigated using a repeated measures analysis of variance (ANOVA) with time (test 1, 2, and 3) as within-subject factor. The assumption of sphericity was checked, and Greenhouse-Geisser correction used in case of violation. The same approach tested differences between time points for the fNIRS outcomes, and post-hoc tests were Bonferroni corrected for multiple comparisons. Next, single and average ICCs, as well as the corresponding confidence intervals (CIs), were calculated using a two-way mixed model with absolute agreement.25,46 Single measure ICCs are reported as the primary measure of test-retest reliability, as only one fNIRS measurement (consisting of seven trials) was performed during each test session.25,46 ICCs were obtained between test 1 and 2 and between test 1 and 3, investigating test-retest reliability on the same day and on consecutive days after cap removal, respectively. ICCs were interpreted as poor (), fair (), good (), or excellent ().25 To allow for a meaningful interpretation, negative ICC values were replaced by zero.25 Additionally, the standard error of measurement (SEM) was calculated as where SDpooled refers to the average standard deviation (SD) across measurements.47,48 Finally, Bland–Altman plots are presented to visualize the mean difference in relation to the average levels of the two tests for each subject separately.493.Results3.1.Participants’ CharacteristicsTwenty-two healthy older adults were recruited for this study, as part of the control group for a larger RCT (clinicaltrial.gov ID: NCT04594148). Two were excluded due to not meeting the in-/exclusion criteria (one had a MoCA and one was aged below 65 years). Participant characteristics of the included 20 healthy older adults are shown in Table 1. They were all right-handed (self-reported). Table 1Participant characteristics.
Note: Normally distributed data are displayed as mean ± SD, and not normally distributed data as median (first quartile - third quartile). MiniBEST, mini balance evaluation systems test; FES-I, Falls Efficacy Scale International; and MoCA, montreal cognitive assessment. MiniBEST, FES-I, and MoCA are presented for descriptive purposes only. 3.2.Behavioral ResultsEven though the postural task was not standardized, weight-shifting performance was similar across the three test sessions, as no differences were found in speed [, , ; see Figure S3(a) in the Supplementary Material] and accuracy [, , , see Figure S3(b) in the Supplementary Material]. Similar results were found for tapping performance [accuracy: , , , see Figure S3(c) in the Supplementary Material; number of taps: , , , see Figure S3(d) in the Supplementary Material]. Exploratory post-hoc tests with Bonferroni corrections for multiple testing also did not show any difference between tests (postural: corrected ; tapping: corrected ). It should be noted, however, that there was a trend towards a better accuracy for tapping in test 2 compared to test 1 (). 3.3.fNIRS ResultsFigure 2 gives an overview of the ICCs and CIs between test 1-2 versus test 1-3 for both and HHb levels. This illustrates that for both outcomes, ICCs were higher for test 1-2 compared to test 1-3 and this particularly in SMA and PMC. The below sections describe these results in more detail. Fig. 2Intraclass correlations (ICC) and CIs of test 1 versus test 2 and test 1 versus test 3 for both (a) oxygenated () and (b) deoxygenated (HHb) hemoglobin. ROIs of the weight-shifting task are displayed with green dot (ICC) and line (CI) and the task-specific ROI of the right finger tapping task (channel C3-C1) are represented by the purple dot (ICC) and line (CI). ![]() 3.3.1.ROI reliability during the postural task without cap repositioningIn the total ROIs (left plus right channels), no differences in were found across time points [PFC: , ; FEFs: , ; SMA: , ; PMC: , ; SSC: , ; see Figs. 3(a)–3(e)]. Exploratory post-hoc tests also did not show differences between the three tests (all corrected ). The test-retest reliability, as captured by the ICC-values and CIs between test 1-2 was excellent for the PFC (, ), PMC (, ) and SSC (, ), and fair for the FEF (, ) and SMA (, ) (see Table 2). Table 2 further illustrates that the reliability for the ROIs per hemisphere showed lower values, but still within an acceptable range (PFC: ; FEF: ; SMA: ; PMC: ; SSC: ). Bland–Altman plots are shown in Fig. 4. Points fell largely within the limits of agreement, were equally distributed around zero, and showed no bias, corroborating the findings above. The pattern, which was overall similar for the different ROIs, did show that one participant with extreme activation (PFC) or deactivation (PMC, SMA) values also had the least consistency between time points. As for the SEMs, values ranged between 1.89 and for the total ROIs and between 2.51 and for the ROIs per hemisphere. Fig. 3(a)–(e) Relative levels (active trial - rest trial) for total (left plus right channels) ROIs at test 1, 2, and 3 for the postural task. Note that the scale on the -axis differs between ROIs as to visualize the slightest differences. (f) Relative levels for all total ROIs averaged over the three test moments. ROI, region of interest; PFC, prefrontal cortex; FEF, frontal eye fields; PMC, premotor cortex; SMA, supplementary motor area; and SSC, somatosensory cortex. ![]() Table 2Test-retest reliability based on single ICCs for relative HbO2 and HHb levels during the postural task.
Note: HbO2, oxygenated hemoglobin; HHb, deoxygenated hemoglobin; ROI, region of interest; SD, standard deviation; ICC, intraclass correlation coefficient; SEM, standard error of measurement; PFC, prefrontal cortex; FEF, frontal eye fields; SMA, supplementary motor area; PMC, premotor cortex; and SSC, somatosensory cortex. Fig. 4Bland–Altman plots for total (left plus right channels) ROIs during the postural task, visualizing the average levels (-axis) and the difference in levels between test 1 and test 2 (-axis). Dotted lines represent the 95% limits of agreement. ![]() Overall, HHb test-retest reliability was lower compared to ( on average for total ROIs), with some exceptions (see Table 2 and Fig. 2). Averaged ICCs, representing the test-retest reliability of the averaged test outcomes50 showed higher values [see Table S2 in the Supplementary Material () and Table S3 in the Supplementary Material (HHb)]. Additionally, individual channel-based analysis revealed that 13 out of 32 channels were activated during the postural weight-shifting task [see Figure S4(a) in the Supplementary Material], including those in the SSC, SMA, and PMC. 3.3.2.ROI reliability during the postural task with cap repositioningTable 2 also illustrates that the repositioning of the cap between test 1 and 3 led to less stable test-retest values than between test 1 and 2. Reliability values were lower for the PFC (, ) and SSC (, ), but remained fair. Reliability became poor for the SMA (, ) and the PMC (, ). However, reliability was good for the FEF (, ). Table 2 also shows that reliability for the ROIs per hemisphere was similar for the motor areas (SMA: ; PMC: ), and lower for the FEF (). Interestingly, ICC-values were higher for the left PFC (, [0.32, 0.85]) and right SSC (, [0.31, 0.85]), but lower for the right PFC (, ) and left SSC (, [0.00, 0.49]). Bland–Altman plots of test 1 and 3 largely confirm the above described results (see Fig. 5). Limits of agreement increased for total ROIs compared to the test 1-2 comparison, especially for the PMC and SMA, but not for the FEF. The same participant with extreme (de)activation as previously mentioned, also showed the least consistency between tests 1 and 3 (PFC, PMC, SMA). SEM scores were also larger between test 1 and 3 (range: ) than between test 1 and 2 (range: ), with the FEF as the only exception (change in SEM: ). SEMs ranged between 3.18 and for the ROIs per hemisphere. Fig. 5Bland–Altman plots for total (left plus right channels) ROIs during the postural task, visualizing the average levels (-axis) and the difference in levels between test 1 and test 3 (-axis). Dotted lines represent the 95% limits of agreement. ![]() The overall HHb test-retest reliability was comparable to ( on average for total ROIs), though less stable between test 1 and 3 than between test 1 and 2 (see Table 2 and Fig. 2). Averaged ICCs showed higher values for both (see Table S2 in the Supplementary Material) and HHb (see Table S3 in the Supplementary Material). 3.3.3.Task-specific fNIRS reliability during finger tappingFor the task-specific motor channel representing the right hand (C3-C1), no differences in relative levels were found across time points during the tapping task [, ; see Fig. 6(a)]. Exploratory post-hoc tests also did not show differences between test moments (all corrected ), even though a trend towards improved tapping accuracy was seen from test 1 to test 2. Interestingly, however, participants who showed better accuracy (lower tapping error) were as stable in their fNIRS outcomes, as assessed with Pearson’s correlations (: , ; HHb: , ; see Figure S5 in the Supplementary Material). The ICC-values were good between tests 1-2 (, ), but became poor between tests 1-3 (, ) (see Table 3). Bland–Altman plots largely confirm these results [see Figs. 6(b) and 6(c)]. Points fell between the limits of agreement, which covered a wider range and were more spread out between test 1 and 3 than between test 1 and 2. In agreement, the SEM score was between test 1 and 2, and between test 1 and 3. Fig. 6(a) Relative levels (active trial - rest trial) at test 1, 2, and 3 for the hand motor channel C3-C1 during the finger tapping task; (b) Bland–Altman plot for channel C3-C1, visualizing the average levels and the difference between levels at test 1 and 2, and (c) test 2 and 3. ![]() Table 3Test-retest reliability based on single ICCs for relative HbO2 and HHb levels during the finger tapping task.
Note: Values are determined for the task-specific motor channel C3-C1. HbO2, oxygenated hemoglobin; HHb, deoxygenated hemoglobin; SD, standard deviation; ICC, intraclass correlation coefficient; and SEM = standard error of measurement. HHb test-retest reliability, as well as the and HHb averaged ICCs (see Table S4 in the Supplementary Material), were better than the single ICCs for , ranging from fair to excellent. Additionally, individual channel-based analysis revealed that five channels of the left hemisphere were active during the right finger-tapping task [see Fig. S4(b) in the Supplementary Material], including the C3-C1 channel, which confirmed the anatomical location of the right-hand motor region. 4.Discussion4.1.Main Study FindingsThis is the first study to investigate fNIRS test-retest reliability of a postural weight-shifting and finger tapping control task in healthy older adults. When performing two measurements with an in-between resting period of 30 min and the cap remained in place, results showed fair (FEF, SMA) to excellent (PFC, PMC, and SSC) reliability during a postural task in five predefined ROIs and good reliability during tapping in one task-specific motor channel. Contrary to our hypothesis, these results are similar to published results in young healthy adults. However, as expected when testing on separate days after removal of the cap, reliability was reduced, and reliability became fair (PFC and SSC) to good (FEF) for the non-motor areas, and poor for the motor areas (SMA, PMC, and C3-C1 hand motor channel). These results are largely in line with previous studies, as detailed in Table 4, with the exception that we found poor reliability in the motor areas. Table 4Comparison between the current work and prior studies on fNIRS test-retest reliability in various motor tasks.
Note: ICC, intraclass correlation coefficient; HbO2, oxygenated hemoglobin; HHb, deoxygenated hemoglobin; and TMS, transcranial magnetic stimulation. Behaviorally, performance on the postural and tapping task did not show significant improvements over time, suggesting that no test-induced learning effects were present. However, a trend towards more accurate tapping was shown from test 1 to test 2, despite providing auditory pacing. Interestingly, this improvement in behavioral accuracy was not associated with a change in fNIRS outcomes. Previous investigations on fNIRS test-retest reliability did not report on the stability of the behavioral correlates.20–24,26,27 Four of these studies (study 1, 2, 5, and 6 in Table 4) standardized performance to some extent to prevent a learning effect, for instance by using cues,22 robotic passive hand movements,21 practicing the task with a metronome,20 or using a very small time-window (1200 ms).24 Furthermore, one study (study 3 in Table 4) checked for possible differences in exhaustion via a Borg scale, showing no differences between test moments.26 4.2.fNIRS Reliability4.2.1.Reliability during postural control without cap removalTest-retest analysis of five predefined and commonly applied ROIs during the postural task revealed an overall fair to excellent reliability in levels when repeating two tests on the same day. This suggests that, when not removing the fNIRS cap and optodes, the fNIRS signal is fairly reliable over time for the FEF and SMA () and highly reliable for the PFC, PMC, and SSC (). Similar results were found for the left and right hemispheres separately. To date, only one other study specifically investigated fNIRS test-retest reliability when testing twice on the same day (study 4 in Table 4),27 although with cap removal. These authors studied only the PFC in young adults, resulting in a good reliability during both straight walking and turning (). The higher reliability found in the current study for the postural task may be explained by not removing the cap, the lack of activity in this area, the shorter duration of signal extraction (20 sec versus 2 min) and higher number of trials (7 versus 1) in which the task was performed.5 Pilot testing prior to data collection also showed a 13% true positive rate increase by adding two trials (67% at five trials and 80% at seven trials). Additionally, other task-related aspects, such as heel strikes during gait, may induce movement artifacts, which were absent during the present postural task. As Stuart et al.27 did not report on HHb, the overall lower reliability compared to found in this study cannot directly be compared. 4.2.2.Reliability during postural control with cap removalAn important finding of the present study is that when measuring fNIRS levels on different days after cap removal and repositioning, reliability deteriorated. Interestingly, non-motor cortical areas seemed less affected by cap removal, indicated by fair to good reliability in the PFC, FEF, and SSC (), which is comparable to the reliability found during walking26 and handgrip exercises23 (study 3 and 7 in Table 4). On the other hand, poor reliability was revealed in the motor areas (), in agreement with a reliability study during passive hand movements21 (study 2 in Table 4), possibly driven by the lack of active movement. The finding that the non-motor areas proved to be more stable across consecutive days could be due the fact that this virtual reality-based postural task required strong non-motor involvement. The relatively poor reliability found for the motor areas during the postural task () is in contrast with previous research on hand grasping and finger tapping, which showed fair to excellent reliability after cap removal () (study 1, 5, and 6 in Table 4).20,22,24 Besides cap repositioning, this finding may alternatively be explained by the fact that the postural task required whole body movements, resulting in wider and more variable cortical recruitment. The single channel-based analysis underscored this statement, as no clear activation pattern was found for the PMC and SMA. In addition, fNIRS signals pertaining to the lower limbs are more prone to systematic artifacts29 and likely more difficult to capture as the motor representation of the lower extremities is located deeply within the interhemispheric fissure,37 which could result in more inconsistent fNIRS measurements. Note that, similar to the present findings, HHb reliability after cap removal was overall comparable to findings in healthy adults (see Table 4). 4.2.3.Task-specific fNIRS reliability during finger tappingFor finger tapping, reliability of the C3-C1 hand motor channel was good when assessed on the same day (). This supports the notion that fNIRS is a sensitive neuroimaging tool for capturing changes during a specific motor tasks requiring localized cortical activation.29 It should be noted that there was a trend towards better behavioral tapping accuracy from test 1 to test 2 (). However, when exploring this result, we did not find differences in the levels or that the participants with improved accuracies had better reliability. Similar to the motor cortical ROIs of the postural task, reliability during tapping became poor when assessed on two consecutive days (), though the decline was less steep ( (SMA) and (PMC) for the postural task and (C3-C1) for the tapping task). This highlights that using fNIRS on 2 consecutive days results in suboptimal reproducibility, despite efforts to standardize cap replacement. In contrast, previous studies investigating task-specific motor areas showed fair to excellent ICC-values () during digit manipulation22 and hand grasping20 when assessed on different days (study 1 and 5 in Table 4). These results, however, were found in young adults, who might show higher signal to noise ratios and therefore higher reliability.28 It could also be that the localization method used in these studies, TMS localization20 and registration of 3D-coordinates,22 provided a more accurate foundation for cap replacement after removal. 4.2.4.Suggestions for improving fNIRS reliabilityFirst, other methods of cap standardization could be explored, such as using a 3D-digitizer, a neuronavigation system51 or structural MRI scans for co-registration.52 Interestingly, we found that averaged ICCs were generally higher than single ICCs.25,46 Especially for study protocols that involve cap removal, multiple fNIRS blocks within one testing session, and the use of averaged values of and HHb levels for between-test comparisons may be required. Second, future studies should investigate whether shortening of trial duration while increasing the number of trials within a block design, could lead to enhanced stability of fNIRS outcomes, as pilot testing revealed increased positive rates using this procedure. Third, behavioral tasks should be standardized as much as possible and any learning effects counteracted, as was done in the present study. Measuring on the same time of day and including large sample sizes is also recommended, especially when using a complex (motor) task. Finally, person-specific variables, such as vigilance, motivation, and effort, could additionally be assessed, as they can possibly affect reliability. 4.3.Strengths and LimitationsDespite a relatively small sample size and the inherently variable nature of fNIRS signals, we found an overall acceptable test-retest reliability of brain oxygenated hemoglobin levels as measured in five commonly used ROIs, as well as in a task-specific motor channel. Although this is the first study investigating test-retest reliability in older adults, future research should investigate whether the findings generalize to patient populations as well. Unlike previous studies, we examined the behavioral test-retest results to take any possible learning effects into account. Moreover, short-separation channels were used to correct for physiological noise in the fNIRS signal.35 A limitation of this study is that we used the same differential path length factor for all participants. It has been shown that age influences this factor during the conversion of optical density into , though it is not known for adults older than 50 years of age.5 Finally, fNIRS measurements are associated with high between-subject variance, as are the outcomes of other neuroimaging techniques, on which the ICC-value is dependent.25 Moreover, the range of CIs accompanying ICCs varied. Therefore, future studies in larger samples are needed to verify fNIRS test-retest reliability in healthy older adults and other study populations. AcknowledgmentsThe authors would like to thank Marie Bogaerts, Annelien Loverix, Hanne Vandenbossche, and Ella Copermans for their help in data collection. This study could not have been done without the contribution of our participants, for which the authors are truly grateful. The work was supported by the European Union’s Horizon 2020 research and innovation programme under the Marie Sklodowska-Curie grant agreement (Grant No. 721577) and the Research Foundation Flanders (FWO) (Grant No. G0A5619N). The funding sources had no involvement in the study design; in the collection, analysis, and interpretation of data; the writing of the report; and in the decision to submit the article for publication. Code, Data, and Materials AvailabilityStudy data and code are not publicly available due to privacy and ethical reasons. Data and code can be made available upon reasonable request to the corresponding author. Supplementary materials are provided online. ReferencesX. Cui, S. Bray and A. L. Reiss,
“Functional near infrared spectroscopy (NIRS) signal improvement based on negative correlation between oxygenated and deoxygenated hemoglobin dynamics,”
Neuroimage, 49
(4), 3039
–3046 https://doi.org/10.1016/j.neuroimage.2009.11.050 NEIMEF 1053-8119
(2010).
Google Scholar
S. C. Bunce et al.,
“Functional near-infrared spectroscopy,”
IEEE Eng. Med. Biol. Mag., 25
(4), 54
–62 https://doi.org/10.1109/MEMB.2006.1657788 IEMBDE 0739-5175
(2006).
Google Scholar
F. Jöbsis,
“Noninvasive, infrared monitoring of cerebral and myocardial oxygen sufficiency and circulatory parameters,”
Science, 198
(4323), 1264
–1267 https://doi.org/10.1126/science.929199 SCIEAS 0036-8075
(1977).
Google Scholar
J. A. Noah et al.,
“fMRI validation of fNIRS measurements during a naturalistic task,”
J. Vis. Exp., 100
(e52116), 5
–9 https://doi.org/10.3791/52116
(2015).
Google Scholar
J. C. Menant et al.,
“A consensus guide to using functional near-infrared spectroscopy in posture and gait research,”
Gait Posture, 82 254
–265 https://doi.org/10.1016/j.gaitpost.2020.09.012
(2020).
Google Scholar
S. Lloyd-Fox, A. Blasi and C. E. Elwell,
“Illuminating the developing brain: the past, present and future of functional near infrared spectroscopy,”
Neurosci. Biobehav. Rev., 34 269
–284 https://doi.org/10.1016/j.neubiorev.2009.07.008 NBREDE 0149-7634
(2010).
Google Scholar
V. Quaresima and M. Ferrari,
“Functional near-infrared spectroscopy (fNIRS) for assessing cerebral cortex function during human behavior in natural/social situations: a concise review,”
Organ. Res. Methods, 22
(1), 46
–68 https://doi.org/10.1177/1094428116658959 1094-4281
(2019).
Google Scholar
F. Herold et al.,
“Functional near-infrared spectroscopy in movement science: a systematic review on cortical activity in postural and walking tasks,”
Neurophotonics, 4
(4), 041403 https://doi.org/10.1117/1.NPh.4.4.041403
(2017).
Google Scholar
Y. Ono et al.,
“Motor learning and modulation of prefrontal cortex: an fNIRS assessment,”
J. Neural Eng., 12
(6), 066004 https://doi.org/10.1088/1741-2560/12/6/066004 1741-2560
(2015).
Google Scholar
M. A. Immink et al.,
“Prefrontal cortex activation during motor sequence learning under interleaved and repetitive practice: a two-channel near-infrared spectroscopy study,”
Front. Hum. Neurosci., 15
(May), 1
–15 https://doi.org/10.3389/fnhum.2021.644968
(2021).
Google Scholar
R. Alves Heinze et al.,
“Hand motor learning in a musical context and prefrontal cortex hemodynamic response: a functional near-infrared spectroscopy (fNIRS) study,”
Cogn. Process., 20 507
–513 https://doi.org/10.1007/s10339-019-00925-y
(2019).
Google Scholar
O. Seidel et al.,
“Motor learning in a complex balance task and associated neuroplasticity: a comparison between endurance athletes and nonathletes,”
J. Neurophysiol., 118
(3), 1849
–1860 https://doi.org/10.1152/jn.00419.2017 JONEA4 0022-3077
(2017).
Google Scholar
M. Hiyamizu et al.,
“Effects of self-action observation on standing balance learning: a change of brain activity detected using functional near-infrared spectroscopy,”
NeuroRehabilitation, 35
(3), 579
–585 https://doi.org/10.3233/NRE-141153
(2014).
Google Scholar
X. Cui et al.,
“A quantitative comparison of NIRS and fMRI across multiple cognitive tasks,”
Neuroimage, 54 2808
–2821 https://doi.org/10.1016/j.neuroimage.2010.10.069 NEIMEF 1053-8119
(2010).
Google Scholar
I. Tachtsidis and F. Scholkmann,
“False positives and false negatives in functional near-infrared spectroscopy : issues, challenges, and the way forward,”
Neurophotonics, 3
(3), 031405 https://doi.org/10.1117/1.NPh.3.3.031405
(2016).
Google Scholar
F. Scholkmann et al.,
“A review on continuous wave functional near-infrared spectroscopy and imaging instrumentation and methodology,”
NeuroImage., 85 6
–27 https://doi.org/10.1016/j.neuroimage.2013.05.004 NEIMEF 1053-8119
(2014).
Google Scholar
H. Niu et al.,
“Test-retest reliability of graph metrics in functional brain networks: a resting-state fNIRS study,”
www.plosone.org
(2013).
Google Scholar
H. Zhang et al.,
“Is resting-state functional connectivity revealed by functional near-infrared spectroscopy test-retest reliable?,”
J. Biomed. Opt., 16
(6), 067008 https://doi.org/10.1117/1.3591020 JBOPFO 1083-3668
(2011).
Google Scholar
H. Zhang et al.,
“Test-retest assessment of independent component analysis-derived resting-state functional connectivity based on functional near-infrared spectroscopy,”
Neuroimage, 55
(2), 607
–615 https://doi.org/10.1016/j.neuroimage.2010.12.007 NEIMEF 1053-8119
(2011).
Google Scholar
D. G. Wyser et al.,
“Characterizing reproducibility of cerebral hemodynamic responses when applying short-channel regression in functional near-infrared spectroscopy,”
Neurophotonics, 9
(1), 015004 https://doi.org/10.1117/1.NPh.9.1.015004
(2022).
Google Scholar
S. Bae, Y. Lee and P. H. Chang,
“There is No test–retest reliability of brain activation induced by robotic passive hand movement: a functional NIRS study,”
Brain Behav., 10
(10), 1
–13 https://doi.org/10.1002/brb3.1788
(2020).
Google Scholar
S. Dravida et al.,
“Comparison of oxyhemoglobin and deoxyhemoglobin signal reliability with and without global mean removal for digit manipulation motor tasks,”
Neurophotonics, 5
(1), 011006 https://doi.org/10.1117/1.NPh.5.1.011006
(2017).
Google Scholar
Y. Bhambhani et al.,
“Reliability of near-infrared spectroscopy measures of cerebral oxygenation and blood volume during handgrip exercise in nondisabled and traumatic brain-injured subjects,”
J. Rehabil. Res. Dev., 43
(7), 845
–856 https://doi.org/10.1682/JRRD.2005.09.0151 JRRDEC 0748-7711
(2006).
Google Scholar
M. M. Plichta et al.,
“Event-related functional near-infrared spectroscopy (fNIRS) based on craniocerebral correlations: reproducibility of activation?,”
Hum. Brain Mapp., 28
(8), 733
–741 https://doi.org/10.1002/hbm.20303 HBRME7 1065-9471
(2007).
Google Scholar
L. Li and Z. Lin,
“Tutorial on use of intraclass correlation coefficients for assessing intertest reliability and its application in functional near-infrared spectroscopy – based brain imaging,”
J. Biomed. Opt., 20
(5), 050801 https://doi.org/10.1117/1.JBO.20.5.050801 JBOPFO 1083-3668
(2022).
Google Scholar
K. C. Broscheid et al.,
“Inter-session reliability of functional near-infrared spectroscopy at the prefrontal cortex while walking in multiple sclerosis,”
Brain Sci., 10
(9), 1
–15 https://doi.org/10.3390/brainsci10090643
(2020).
Google Scholar
S. Stuart et al.,
“Pre-frontal cortical activity during walking and turning is reliable and differentiates across young, older adults and people with Parkinson’s disease,”
Front. Neurol., 10
(MAY), 1
–11 https://doi.org/10.3389/fneur.2019.00536
(2019).
Google Scholar
M. Dadar, V. S. Fonov and D. L. Collins,
“A comparison of publicly available linear MRI stereotaxic registration techniques,”
Neuroimage, 174 191
–200 https://doi.org/10.1016/j.neuroimage.2018.03.025 NEIMEF 1053-8119
(2018).
Google Scholar
H. Cockx et al.,
“Functional near-infrared spectroscopy is sensitive to leg activity in the primary motor cortex, but requires rigorous correction for systemic fluctuations induced by movements,”
in OSF Prepr.,
1
–35
(2022). https://doi.org/10.31219/osf.io/xgnfh Google Scholar
J. Willaert et al.,
“Does a novel exergame challenge balance and activate muscles more than existing off-the-shelf exergames?,”
J. Neuroeng. Rehabil., 3
(1), 1
–13 https://doi.org/10.1186/s12984-019-0628-3
(2020).
Google Scholar
V. de Rond et al.,
“Compromised brain activity with age during a game-like dynamic balance task: single- vs. dual-task performance,”
Front. Aging Neurosci., 13
(July), 1
–13 https://doi.org/10.3389/fnagi.2021.657308
(2021).
Google Scholar
D. A. Winter,
“Biomechanics and Motor Control of Human Movement,”
John Wiley & Sons, Inc.(
(2009). Google Scholar
C. Stamate et al.,
“The cloudUPDRS app: a medical device for the clinical assessment of Parkinson’s disease,”
Pervasive Mob. Comput., 43 146
–166 https://doi.org/10.1016/j.pmcj.2017.12.005
(2018).
Google Scholar
G. Augusto et al.,
“fNIRS optodes’ location decider (fOLD): a toolbox for probe arrangement guided by brain regions-of-interest OPEN,”
Sci. Rep., 8 3341 https://doi.org/10.1038/s41598-018-21716-z SRCEC3 2045-2322
(2018).
Google Scholar
H. Santosa et al.,
“Quantitative comparison of correction techniques for removing systemic physiological signal in functional near-infrared spectroscopy studies,”
Neurophotonics, 7
(3), 035009 https://doi.org/10.1117/1.NPh.7.3.035009
(2020).
Google Scholar
V. Jurcak, D. Tsuzuki and I. Dan,
“10/20, 10/10, and 10/5 systems revisited: their validity as relative head-surface-based positioning systems,”
Neuroimage, 34 1600
–1611 https://doi.org/10.1016/j.neuroimage.2006.09.024 NEIMEF 1053-8119
(2007).
Google Scholar
W. Penfield and E. Boldrey,
“Somatic motor and sensory representation in the cerebral cortex of man as studies by electrical stimulation,”
Brain, 60
(4), 389
–443 https://doi.org/10.1093/brain/60.4.389 BRAIAK 0006-8950
(1937).
Google Scholar
H. Santosa et al.,
“The NIRS brain AnalyzIR toolbox,”
Algorithms, 11
(5), 73 https://doi.org/10.3390/a11050073 1748-7188
(2018).
Google Scholar
G. Strangman, M. A. Franceschini and D. A. Boas,
“Factors affecting the accuracy of near-infrared spectroscopy concentration calculations for focal changes in oxygenation parameters,”
Neuroimage, 18 865
–879 https://doi.org/10.1016/S1053-8119(03)00021-1 NEIMEF 1053-8119
(2003).
Google Scholar
R. D. Hoge et al.,
“Simultaneous recording of task-induced changes in blood oxygenation, volume, and flow using diffuse optical imaging and arterial spin-labeling MRI,”
Neuroimage, 25
(3), 701
–707 https://doi.org/10.1016/j.neuroimage.2004.12.032 NEIMEF 1053-8119
(2005).
Google Scholar
J. W. Barker, A. Aarabi and T. J. Huppert,
“Autoregressive model based algorithm for correcting motion and serially correlated errors in fNIRS,”
Biomed. Opt. Express, 4
(8), 1366 https://doi.org/10.1364/BOE.4.001366 BOEICL 2156-7085
(2013).
Google Scholar
J. Virtanen et al.,
“Accelerometer-based method for correcting signal baseline changes caused by motion artifacts in medical near-infrared spectroscopy,”
J. Biomed. Opt., 16
(8), 087005 https://doi.org/10.1117/1.3606576 JBOPFO 1083-3668
(2011).
Google Scholar
P. H. S. Pelicioni et al.,
“Cortical activation during gait adaptability in people with Parkinson’s disease,”
Gait Posture, 91 247
–253 https://doi.org/10.1016/j.gaitpost.2021.10.038
(2022).
Google Scholar
M. A. Mayka et al.,
“Three-dimensional locations and boundaries of motor and premotor cortices as defined by functional brain imaging: a meta-analysis,”
Neuroimage, 31
(4), 1453
–1474 https://doi.org/10.1016/j.neuroimage.2006.02.004 NEIMEF 1053-8119
(2006).
Google Scholar
V. Letchuman and C. Donohoe, Neuroanatomy, Superior Sagittal Sinus, StatPearls, Treasure Island(
(2019). Google Scholar
T. K. Koo and M. Y. Li,
“A guideline of selecting and reporting intraclass correlation coefficients for reliability research,”
J. Chiropr. Med., 15
(2), 155 https://doi.org/10.1016/j.jcm.2016.02.012
(2016).
Google Scholar
J. P. Weir,
“Quantifying test-retest reliability using the intraclas correlation coefficient and the SEM,”
J. Strength Cond. Res., 19
(1), 231
–240 https://doi.org/10.1519/15184.1
(2005).
Google Scholar
C. Strouwen et al.,
“Test-retest reliability of dual-task outcome measures in people with parkinson disease,”
Phys. Ther., 96
(8), 1276
–1286 https://doi.org/10.2522/ptj.20150244 POTPDY
(2016).
Google Scholar
J. Martin Bland and D. G. Altman,
“Statistical methods for assessing agreement between two methods of clinical measurement,”
Lancet, 327
(8476), 307
–310 https://doi.org/10.1016/S0140-6736(86)90837-8 LANCAO 0140-6736
(1986).
Google Scholar
T. Johnstone et al.,
“Stability of amygdala BOLD response to fearful faces over multiple scan sessions,”
Neuroimage, 25
(4), 1112
–1123 https://doi.org/10.1016/j.neuroimage.2004.12.016 NEIMEF 1053-8119
(2005).
Google Scholar
S. L. Novi et al.,
“Integration of spatial information increases reproducibility in functional near-infrared spectroscopy,”
Front. Neurosci., 14 746 https://doi.org/10.3389/fnins.2020.00746 1662-453X
(2020).
Google Scholar
M. A. Yücel et al.,
“Best practices for fNIRS publications,”
Neurophotonics, 8
(1), 1
–34 https://doi.org/10.1117/1.NPh.8.1.012101
(2021).
Google Scholar
BiographyVeerle de Rond is a PhD candidate at Neurorehabilitation Research Group (eNRGy) at KU Leuven. She obtained her BSc and MSc degrees in human movement sciences at the University of Groningen. Her research interests include the behavioral, neural (fNIRS), and neuromuscular (EMG) correlates of postural control and motor learning in ageing. Moran Gilat is an assistant professor at the Parkinson Rehabilitation Research Lab (PRO-labo) within the Neurorehabilitation Research Group (eNRGy) at KU Leuven. His research focuses on the assessment, prediction, and treatment of freezing of gait in patients with Parkinson’s disease. Additionally, he is interested in developing interventions that improve motor learning and the accompanied neuroplasticity (fMRI, EEG, and fNIRS) in Parkinson’s disease. Nicholas D’Cruz is a physical therapist and postdoctoral fellow at the Parkinson Rehabilitation Research Lab within the Neurorehabilitation Research Group and at the Motor Control and Neuroplasticity Research Group at KU Leuven. His research focuses on the behavioral and neural correlates (fMRI and fNIRS) of freezing of gait in patients with Parkinson’s disease and the effects of split-belt training. Femke Hulzinga is a PhD candidate at the Parkinson Rehabilitation Research Lab within the Neurorehabilitation Research Group at KU Leuven. She obtained her BSc degree in human movement sciences at VU University Amsterdam and her MSc degree in biomedical sciences at Radboud University Nijmegen. Her research mainly focuses on split-belt training as a potential tool for improving gait in Parkinson’s disease. She is also interested in the underlying neural (fNIRS) and neuromuscular mechanisms. Jean-Jacques Orban de Xivry is a full professor at the Motor Control and Neuroplasticity Research Group at KU Leuven. He is an engineer in applied mathematics by background and earned his PhD on computational neuroscience. His research interests include understanding the neural control (EEG and fNIRS) of movement and motor learning, primarily in the context of ageing and age-related diseases. Alice Nieuwboer is a full professor at the Parkinson Rehabilitation Research Lab at KU Leuven. She is a physiotherapist by background and made several original contributions to gait and motor control research in Parkinson’s disease (PD), more specifically on freezing of gait. She oversees several clinical trials, investigating whether cueing and split-belt training are effective and whether consolidated motor learning can be achieved in PD and how this imprints on the brain. |
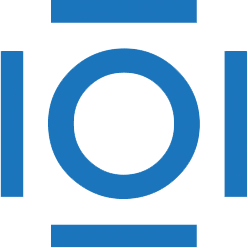
CITATIONS
Cited by 3 scholarly publications.
Reliability
Shape memory alloys
Near infrared spectroscopy
Neurophotonics
Brain
Visualization
Hemodynamics