|
ACCESS THE FULL ARTICLE
No SPIE Account? Create one
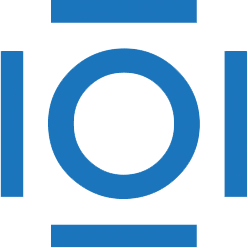
CITATIONS
Cited by 5 scholarly publications.
Inspection
LCDs
Digital image correlation
Optical inspection
Neural networks
Imaging systems
Optical engineering