Existing roughness machine vision inspection methods based on designed indices require the artificial construction of features associated with roughness based on image information. Such methods are less universal but highly demanding for the imaging environment and cannot be directly applied to general industrial environments. The roughness machine vision inspection methods based on traditional deep learning, on the other hand, rely heavily on a large number of training samples, and the model needs to be retrained when the recognition of new categories is required by the inspection task. Therefore, a machine vision inspection method for milling surface roughness grade based on the improved model-agnostic meta-learning (MAML++) network was proposed to address the above problems. This method could not only automatically extract the image features associated with roughness but also recognize the surface roughness grade of milling workpieces by a small number of labeled samples. It reduced the need for sample size and can recognize new categories without retraining the model. The experimental results showed that the method exhibited high accuracy in roughness inspection tasks with different sample distributions and had promising robustness to complex light environments. In addition, in terms of the requirement for recognizing new roughness categories, a low-cost and fast roughness inspection task could be accomplished by acquiring fewer labeled samples. In summary, the proposed model has the potential to be a fast inspection system that can be applied to surface roughness inspection for most industrial applications. It provides the technology for inline inspection of machine vision roughness in general industrial lighting environments. |
ACCESS THE FULL ARTICLE
No SPIE Account? Create one
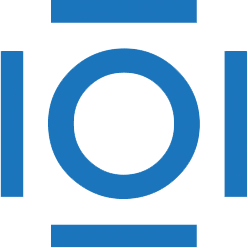
CITATIONS
Cited by 3 scholarly publications.
Inspection
Education and training
Surface roughness
Visual process modeling
Machine vision
Data modeling
Statistical modeling