Phase unwrapping is an indispensable step for many optical imaging and metrology techniques. The rapid development of deep learning has brought ideas to phase unwrapping. In the past four years, various phase dataset generation methods and deep-learning-involved spatial phase unwrapping methods have emerged quickly. However, these methods were proposed and analyzed individually, using different strategies, neural networks, and datasets, and applied to different scenarios. It is thus necessary to do a detailed comparison of these deep-learning-involved methods and the traditional methods in the same context. We first divide the phase dataset generation methods into random matrix enlargement, Gauss matrix superposition, and Zernike polynomials superposition, and then divide the deep-learning-involved phase unwrapping methods into deep-learning-performed regression, deep-learning-performed wrap count, and deep-learning-assisted denoising. For the phase dataset generation methods, the richness of the datasets and the generalization capabilities of the trained networks are compared in detail. In addition, the deep-learning-involved methods are analyzed and compared with the traditional methods in ideal, noisy, discontinuous, and aliasing cases. Finally, we give suggestions on the best methods for different situations and propose the potential development direction for the dataset generation method, neural network structure, generalization ability enhancement, and neural network training strategy for the deep-learning-involved spatial phase unwrapping methods. |
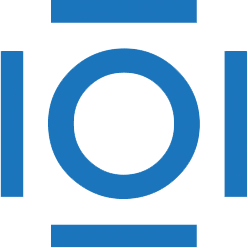
CITATIONS
Cited by 52 scholarly publications.
Neural networks
Signal to noise ratio
Photonics
Superposition
Denoising
Zernike polynomials
Computer simulations