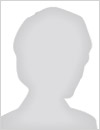
This will count as one of your downloads.
You will have access to both the presentation and article (if available).
Texture classification of anatomical structures in CT using a context-free machine learning approach
The ImageCLEFmed benchmark proposes a medical case-based retrieval task. This task aims at retrieving articles from the biomedical literature that are relevant for differential diagnosis of query cases including a textual description and several images. In the context of this campaign many approaches have been investigated showing that the fusion of visual and text information can improve the precision of the retrieval. However, fusion does not always lead to better results.
In this paper, a new query-adaptive fusion criterion to decide when to use multi-modal (text and visual) or only text approaches is presented. The proposed method integrates text information contained in MeSH (Medical Subject Headings) terms extracted and visual features of the images to find synonym relations between them. Given a text query, the query-adaptive fusion criterion decides when it is suitable to also use visual information for the retrieval.
Results show that this approach can decide if a text or multi{modal approach should be used with 77.15% of accuracy.
Using heterogeneous annotation and visual information for the benchmarking of image retrieval system
View contact details
No SPIE Account? Create one